How tech will shape biotech in 2024
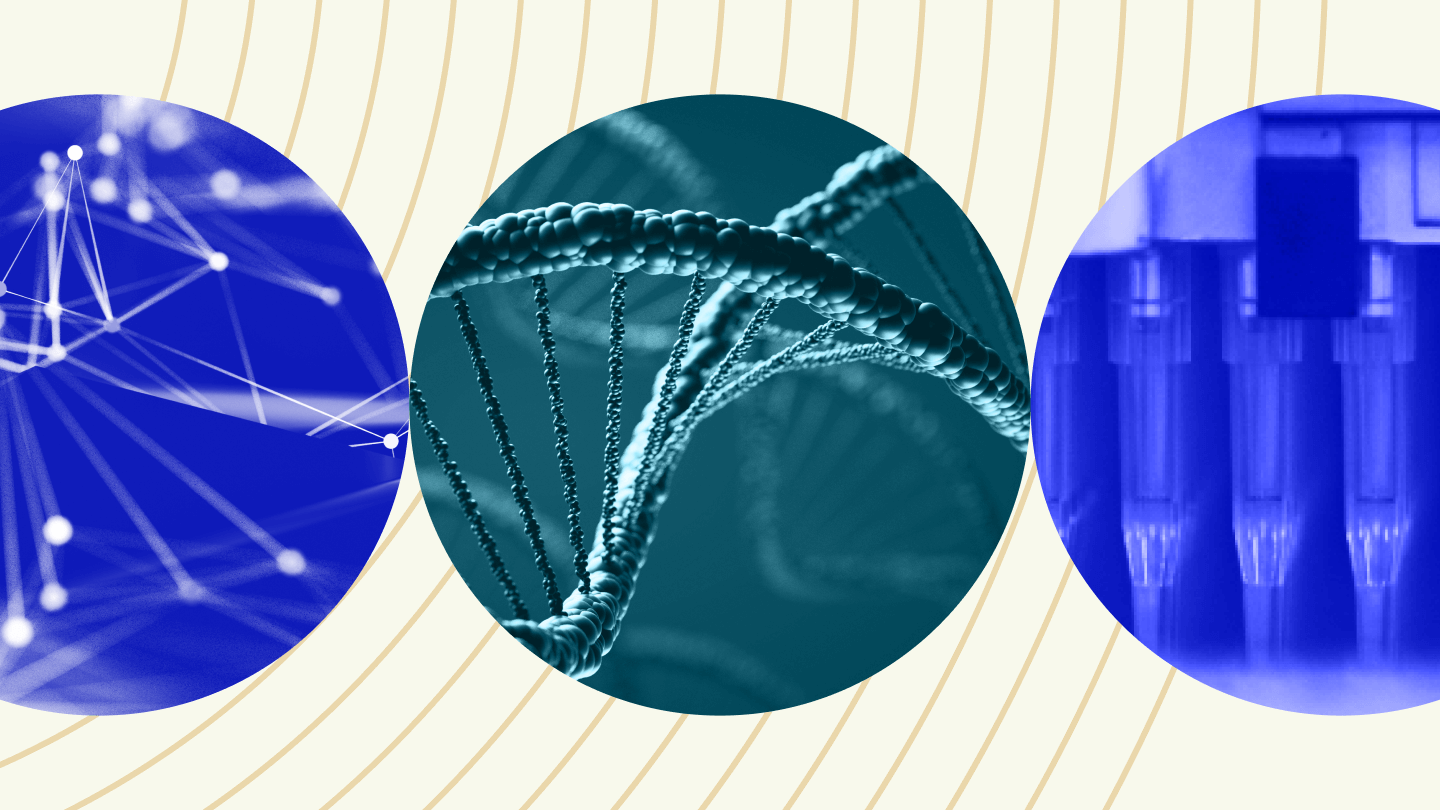
We’re in a new era of biotech, defined by the interplay between biology, data and AI. And it's the enabling technologies — the connected lab instruments, cloud-based scientific applications, R&D data platforms, and AI tools — that are becoming the focus of ‘tech’ in biotech. These technologies are now inextricably linked to efforts to rapidly understand and successfully engineer biology, to drive scale, innovation, and improve margins. A biotech’s tech strategy is now its competitive edge.
Where will these technologies and the biotech community focus and drive innovation in the year ahead? Janet Matsen, PhD, a software engineer focused on machine learning at Benchling, and Vega Shah, PhD, a product marketing manager focused on research, weigh in on their predictions for how tech will shape biotech for the year ahead.
Commercial progress with AI in biotech will be slower than in tech, but in the long-term more transformational.
Why does biotech AI/ML move slower than tech? Tech has the luxury of easier data modeling, easier featurization, and incredible volumes of data. Biotech, on the other hand, is challenged by all three factors.
AI for biotech requires large-scale, standardized, and very expensive data, in addition to a mathematical representation of both the physical matter being optimized. While most biotechs have a good conceptual sense of how AI/ML can help them, they need better metadata, larger volumes of data, the expertise to apply the right model to the problem, and the skill to troubleshoot root causes when results are poor.
Say you want to optimize strains, for example. You can spend a lot of time wrestling with concepts to establish a meaningful tabular representation of those genomes. You also need a physical genetic engineering workshop to build genetic variants, and a bioinformatics pipeline to populate your data model. You end up with data points (rows) of data that are orders of magnitude more expensive than data you’d use in most other technology businesses. This constrains the volume of data you can collect, and makes it more difficult for algorithms to find patterns among noise.
We won’t get the same instant gratification with AI/ML that is often seen in tech. But ultimately, AI/ML will help the industry to program therapeutics; it won’t just be about discovery. AI/ML in biotech will deliver products that transform the world and make lives better: just look at Insitro’s progress in applying ML to discover new treatments for ALS.
This year, companies will focus on the ‘building-the-foundations’ stage to make AI/ML possible. This includes the systems to standardize and structure data, cultivating talent and the right skills, doing the hard work of cleaning data amongst all of biology’s curveballs. Creating data that’s fit for AI/ML will be a critical differentiator for success — and it will be worth the investment.
LLMs will boost productivity and creativity for scientists, just as they have for engineers.
Odds are you have a computational colleague who would be giddy to tell you a story about how they worked faster and better with ChatGPT or GitHub CoPilot.
Scientists will start enjoying the same productivity and creativity boosts as they lean into using AI chatbots. LLM-savvy scientists will begin discussing experimental plans with chatbots, asking them to help identify sources of error, propose metadata they should track, suggest appropriate statistical tests for the data they generate, and reformat and summarize data from experiments, saving hours/days of legwork. OpenAI’s Code Interpreter and Benchling’s own Report and Chart Generation with LLMs are all good ones to start with.
For scientists, AI bots should be thought of as muses, not oracles. Of course, this technology is imperfect, and can reply with incorrect or incomplete information. But so, too, can your colleagues. Do what computational folks are doing: iteratively refine your prompt, and think of the output as a brainstorm rather than an expert opinion.
Building the data foundations, systems, and teams to set up for custom in-house AI is usually a longer-term investment, but that doesn’t mean scientists can’t be using and experimenting with LLMs today.
The tipping point is here for advanced modalities, and it will drive an R&D IT reset.
The FDA approved almost 50% more novel drugs in 2023 than in 2022, marking the second highest count in the past 30 years, and providing us with breakthrough therapies for obesity, Alzheimer's, and sickle cell disease.
It’s not just about volume, but also the type. The number of advanced modalities and biologic approvals is rising. With the pending patent cliff that puts tens of billions of dollars in loss of exclusivity risk, companies are looking for their next blockbuster. Precision medicines, new GLP-1 treatments — differentiated products with better patient outcomes set companies up to scale the patent cliff.
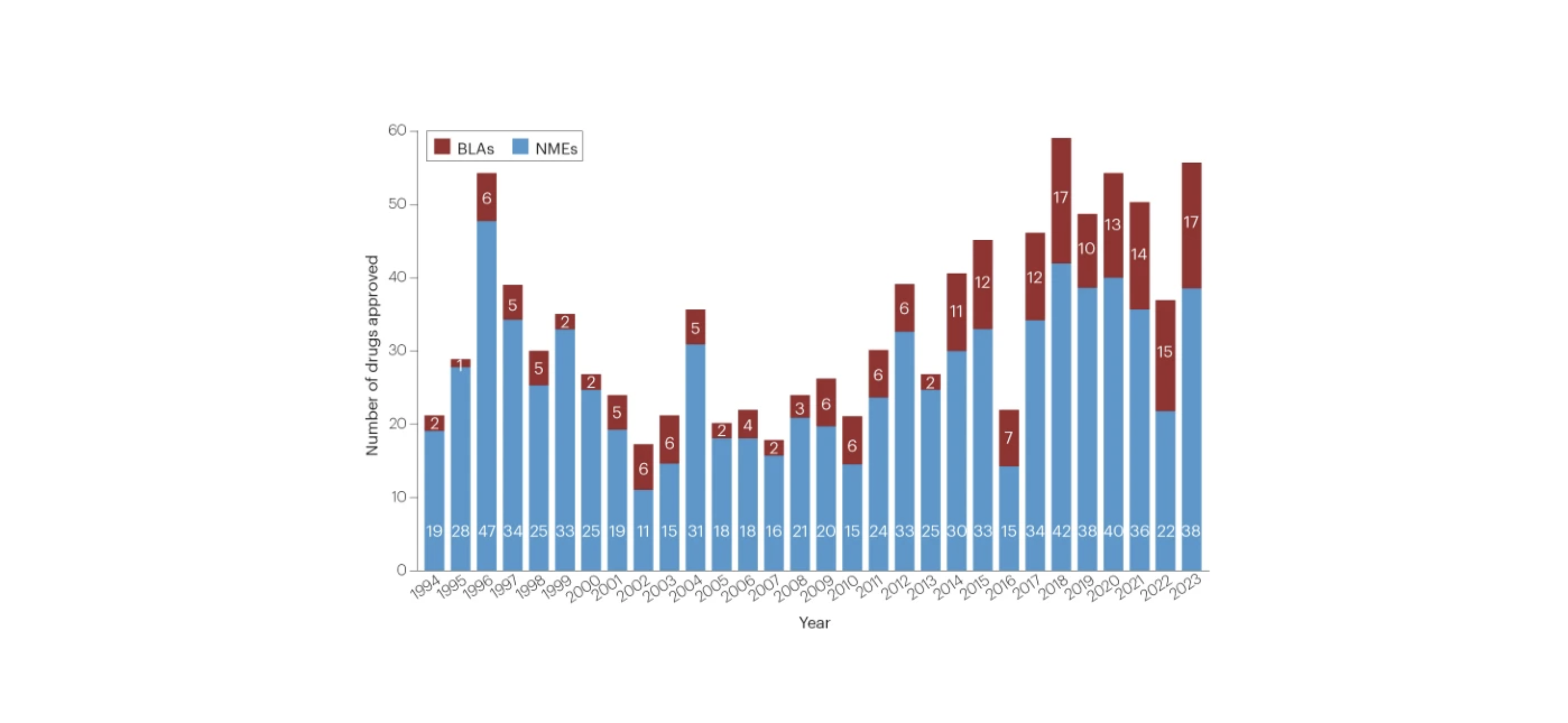
Figure 1: Annual FDA approvals for new molecular entities (NMEs) and biologics license applications (BLAs) over the last 30 years. (Source: Nature).
This shift to new modalities is a strategic inflection point, requiring biopharma to rethink their operations. New modalities upend the way work is done in the lab, with growing needs to process high volumes of complex, multimodal data in collaboration with multiple stakeholders. Legacy tools built for small molecule R&D are no longer fit for purpose here.
Scientists require more flexible data models which support the molecular complexity as well as the rate of change in experimental techniques being used. Flexible data models also need to meet the business needs to obtain compliance-friendly data. Closer collaboration between research and development teams has become an imperative: teams need greater visibility and access to key pieces of the underlying technology to bring products to market, this visibility is now mandatory early and throughout the R&D lifecycle.
The software that scientists rely on to do their R&D needs to account for these big shifts, and will also be seen as the key to unlocking the opportunity with new modalities. This year, we’ll see more emphasis on end-to-end R&D software that allows scientists to follow the molecule across teams and stages, and more emphasis on flexible data models and automation of workflow. The companies that are able to adjust their IT and systems to incorporate advanced modalities will be set up for decades to come.
Biotech community will grow in force and be a catalyst for industry change.
Biotech is going through a renaissance, with the rise of new modalities and an increasingly technical lab. With this, the industry needs to evolve the tools it uses (see above prediction), how it operates, who it hires, and how it communicates. One of the major forces that’s pushing these changes along is entirely grassroots: community.
Communities have existed in life sciences for decades. But today, it’s not just that the numbers are growing in forums, online, and in-person — behaviors are also changing. In any of these communities, be it BitsinBio, BIOS, Nucleate, or ML4ProteinEngineering, people crowdsource technical knowledge and expert opinion of the hivemind, they share lessons learned and mentorship. We see this firsthand in the Benchling Community as well.
This shift may sound trivial. But biotech has a culture that’s rooted in protecting IP, in safeguarding privacy. Opacity trumped transparency. Contrast that to what’s going on in these communities today, where sharing business, management, and bioinformatics tips is the norm. This is a big departure. And this will be a driving force in the digital transformation of biotech. Scientists don’t share their proprietary science, instead the conversation is about new technologies, data models, breakthroughs with computational systems that they’re building.
These communities will also be a driving force in attracting technical and diverse talent and in embracing outsiders. ‘No, you don’t need a bio background’ is a common refrain. Newcomers get the support, resources, and inspiration they need to make the leap into biotech.
We’re obviously biased, but biotech is the industry where we need to attract more talent, excite minds, and share best practices and learnings, as we work to develop better cures and more sustainable products. These communities will have outsized impact in the year ahead, doing a lot of the heavy lifting of bringing in the necessary new tools and talent, opening access, and solving tough problems collectively.
Learn more about AI experimentation at Benchling
See how Benchling is using generative AI today and get resources on getting started with LLMs in biotech.
Powering breakthroughs for over 1,200 biotechnology companies, from startups to Fortune 500s