An Interdisciplinary, Data-Driven Approach to Re-Engineering Orthogonal Riboswitches for Enhanced Function
This article was contributed by:
Ross Kent
Graduate Student, Dixon LabUniversity of Manchester Follow Ross on Twitter at @rwk202 and the Dixon Lab at @BiotechDixon.
Riboswitches: Function and Application
Traditional tools for regulating gene expression generally utilise allosteric transcription factors. More recently, RNA regulatory devices have gained significant attention as novel tools for regulating transcription and translation. One such class of RNA-mediated devices are riboswitches. Riboswitches are structured RNA sequences, commonly found in the 5' UTR of bacterial genes. Following transcription, the 5' UTR of the RNA molecule folds into a specific secondary structure, which is able to recognise and bind to a specific small-molecule inducer. This ultimately alters protein production.
Riboswitches are attractive regulatory tools due to their small size, cis-acting regulatory function and ligand specificity. We can harness these natural tools for post-transcriptional regulation in bacteria. By decoupling transcription and translation in the absence of riboswitch induction, we are able to dramatically reducing basal levels of protein production. Riboswitch regulation allows more precise regulation of protein production. By tuning the rate of gene expression, we can slow down protein production and reduce the burden of overexpression within the host cell. Riboswitches can also be applied broadly, including the manipulation endogenous gene expression to study gene function and the bio-sensing of small molecules. But riboswitch technology is particularly useful for the heterologous production of proteins that are toxic, that are difficult to express, or that synthesise toxic products or intermediates.
Orthogonal Regulation of Bacterial Gene Expression
In the Dixon Lab, based at the University of Manchester - Manchester Institute of Biotechnology, we work on translation-activating riboswitches. These switches function by sequestering the ribosome-binding site (RBS) within a complex secondary structure. In the absence of the ligand, the RBS is folded within a hairpin structure, and this “OFF” state causes repression of translation initiation. Upon ligand binding, the RNA undergoes a structural rearrangement to an “ON” state, leading to the release of the ribosome binding site and allowing the ribosome to initiate translation. See Figure 1A for a schematic representation of the function of translation-activating riboswitches.
Our lab focuses on a synthetic orthogonal riboswitch (ORS), which responds to a synthetic analogue of adenine called pyrimido[4,5-d]pyrimidine- 2,4-diamine (PPDA). This riboswitch was previously engineered by mutagenesis from a natural adenine riboswitch to alter the ligand specificity.1–3 The modified riboswitch allows us to regulate gene expression by titration of PPDA. Unlike adenine, PPDA is not metabolised.4 As a result, we can precisely and predictably tune the rate of translation in an orthogonal manner. We aim to understand how these regulatory devices can be systematically engineered to further improve their functions.
There are a number of significant challenges that prevent us from using riboswitches in a "plug and play" manner. Firstly, riboswitch function is highly sensitive to sequence context; changing the protein coding sequence downstream of the riboswitch can negatively impact riboswitch function. This lack of modularity makes it challenging for other researchers to easily use our riboswitches in their own research and to regulate a different gene. Secondly, the maximum level of expression and dynamic range of many riboswitches is often quite modest.
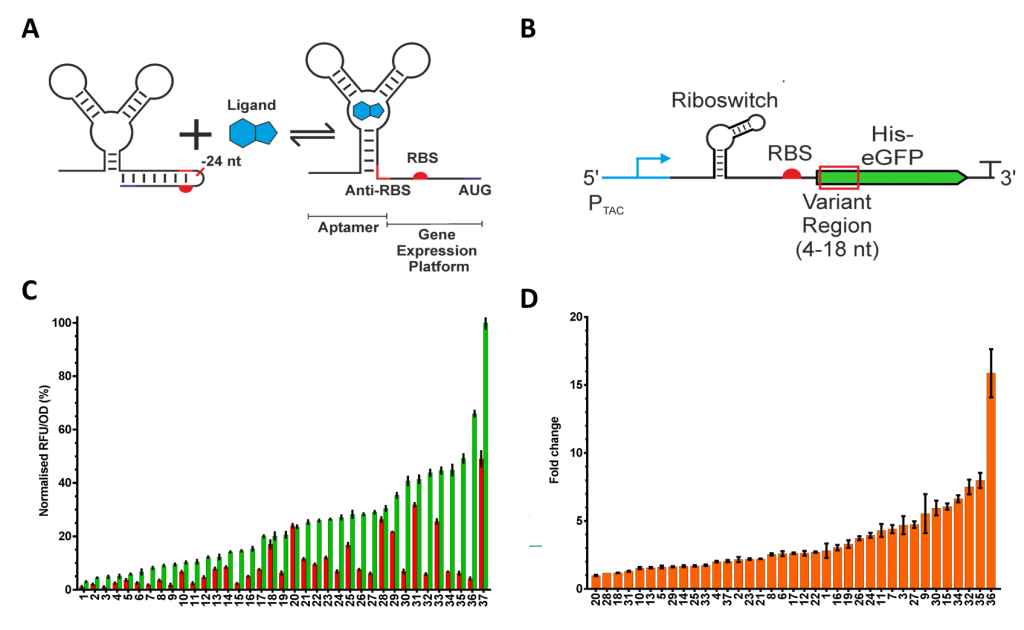
Figure 1. Riboswitch function, context sensitivity and codon optimisation. (A) Translation initiation regulating riboswitches function by modulating access to the RBS. In the case of the ORS, the binding of PPDA causes the RBS to be released from the anti-RBS-RBS hairpin through a structural rearrangement of the expression platform. (B) The function of the riboswitch is sensitive to changes in the surrounding sequence. To explore this we changed the codon usage of the N-terminal region (+4 nt - +18 nt relative to the adenine residue of the start codon), highlighted in red. These codon variants were placed under the control of an IPTG inducible PTAC promoter and the ORS. (C) From the variant libraries, 37 functional riboswitches were isolated and with an “OFF” range (red), “ON” range (green), and (D) dynamic range.
Complex Methods for Tackling Context Sensitivity and Performance Limitations
We sought to understand the context sensitivity by changing the transcript sequence associated with the riboswitch. To do so, we modified codon usage of an N-terminal region of 6xHis insulated eGFP, (Figure 1B). We were able to identify variants with a wide range of functionality (Figure 1C and 1D), highlighting the impact even small sequence changes can have on riboswitch performance.5
The mRNA for each codon variant was sequenced and characterised according to a number of different attributes, including codon usage bias, predicted translation initiation rate, and GC content. We predicted minimum free energies of the OFF state (ΔG Full), ON state (ΔG Trunc) and the switching energy (ΔΔG = ΔG Full - ΔG Trunc). Correlation analysis of the relationship between in silico calculated sequence characteristics and in vivo performance of three aspects of riboswitch function, OFF and ON expression and the dynamic range, revealed a number of interesting relationships (explained in Figures 2A-D). Most interestingly, we observed three clusters of riboswitch function, when we compared ΔΔG and dynamic range (Figure 2E) highlighting a “sweet spot” where ΔΔG and dynamic range gave optimal performance.
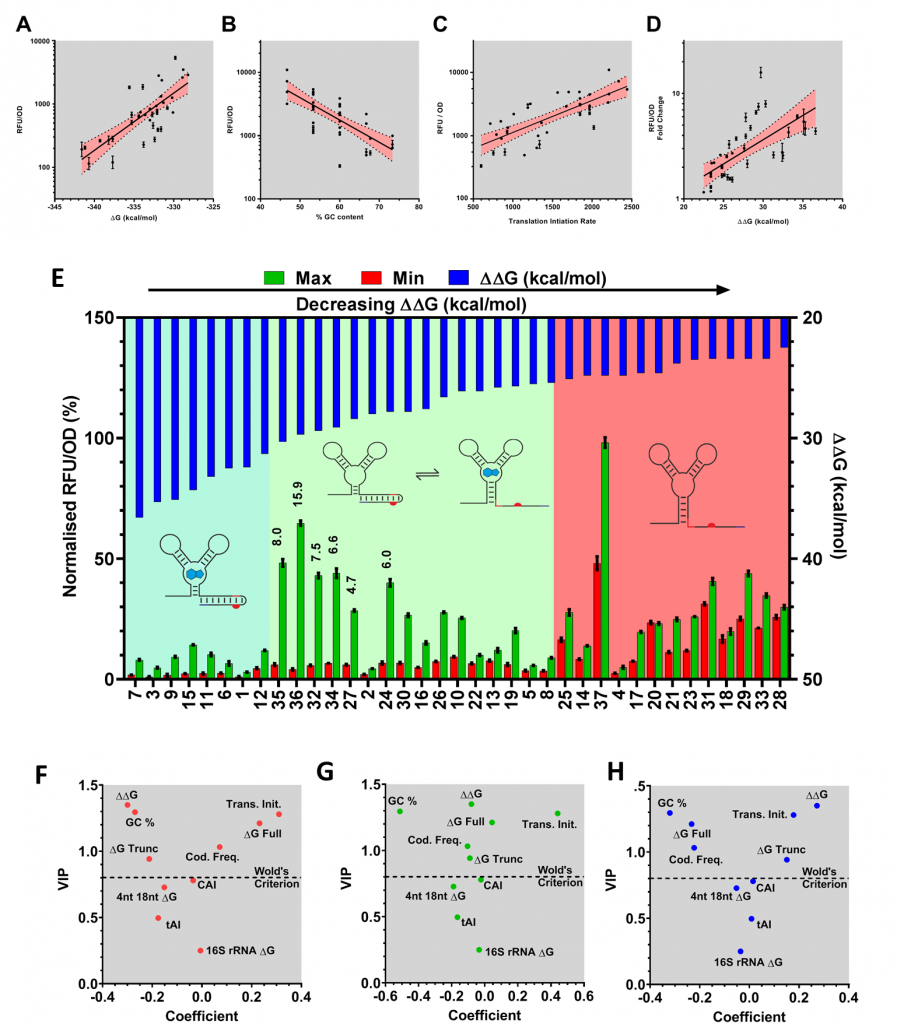
Figure 2. Understanding context sensitivity through PLS regression. Pearson linear regression highlights correlations between (A) ΔG and OFF expression, (B) GC content and ON, (C) T.I.R, and (D) ON and ΔΔG with dynamic range. (E) One key observation following investigation of the was that codon usage variant can be classified into three clusters according to the calculated ΔΔG. These clusters are always OFF (blue box, 7 variants), functional (white box, 16 variants), and always ON (yellow box, 13 variants) where switches are very leaky and regulatory function is less than 2-fold. The best performing riboswitches are annotated with their respective dynamic range performance (35, 36, 32, 34, 27 and 30). PLS modeling of the sequence-function relationships allowed further exploration of these complex relationships. The importance of the analysed sequence characteristics in predicting OFF (F), ON (G) and fold change (H) was assessed through analysis of model coefficients and VIP (variable importance in projection) values.
To investigate the relationships between the N-terminal sequence and in vivo riboswitch performance, we employed a correlation analysis and supervised learning method called Partial Least Squares (PLS). PLS uses latent variables to reduce dimensionality and explain covariance between a set of factors and responses. This methodology is particularly useful when modeling datasets with a high number of collinear variables. Through this approach, we can begin to unravel the complex relationships between each of our sequence characteristics and riboswitch function (Figure 2).
Design of Experiments and Expression Platform Engineering
Once we had improved our understanding of the context sensitivity of the ORS, we set out to improve the dynamic range and maximal ON expression level. To enhance performance, we re-engineered the expression platform of the ORS (Figure 1A) by replacing the weak native RBS (AGAGAA) with a stronger one (AGGAGG).8 The RBS is involved in the structural conformation of the expression platform, so the corresponding anti-RBS of the OFF structure needed to be compatible. With two rounds of Fluorescence Associated Cells Sorting (FACS), we screened a library of expression platform variants and were able to isolate functional riboswitches with dramatically increased expression and dynamic range.
We next sought to combine the improved expression platform variants with the best performing N-terminal 6x His tag insulator regions. In addition to testing different combinations of genetic parts, we were keen to select a final riboswitch that would perform robustly in a wide array of expression conditions. Fully characterising this highly dimensional experimental space would require repetitive, costly cloning and time-consuming experimental effort. Therefore, to carry out this mapping efficiently, we applied a Design of Experiments (DoE) approach. DoE is a statistical method for the systematic exploration of highly dimensional factor space. This approach facilitates simultaneous, multi-factorial optimisation of complex processes using structured experimental design. It enables us to drastically reduce the number of experiments required to map the factor space and to understand a large number of factors and their interactions. We reduce experimental burden, thereby saving time and money.
By understanding how experimental factors interact, we can make a more informed design choice and select parts with minimal interactions. In our research, an interaction between two genetic factors indicates sequence-context sensitivity, and a genetic-environment interaction implies poor robustness.
We used this DoE approach to explore the relationships between RBS strength, N-terminal linker, anti-RBS, induction temperature and transcriptional induction (IPTG concentration) (Figure 3A). We explored the impact of these characteristics on four responses: basal expression, maximal expression, riboswitch dependent fold change and total fold change. Testing all possible combinations of these factors using traditional experimentation would require 216 experimental runs. Through DoE, we are able to do this in just 40. We could identify the conditions that gave drastically increased performance and interrogate the complex effects and interactions underlying this improved performance. Following quantification of eGFP expression when uninduced, OFF (induced with IPTG only), and ON (induced with IPTG and PPDA), we were able to observe high levels of diversity in riboswitch function between each of the experimental conditions specified by the DoE design (Figure 3B).
Interestingly, we identified an interaction between the N-terminal linker and the induction temperature. The modeling predicted that riboswitches containing the L36 His tag linker variant (Figure 3C) would show reduced fold-change performance at 37 °C compared to those containing the L35 linker (Figure 3D). This prediction was experimentally validated by measuring eGFP production when uninduced, OFF, and ON (Figure 3E). The optimal riboswitch device enabled riboswitch-mediated regulation of expression across a 72-fold dynamic range compared to induction with IPTG only, and 550-fold over the basal level of expression (Figure 3F). Through this insight we were able to select a final device that did not show an interaction with temperature, giving more robust and predictable performance across different expression conditions. Had we employed a traditional One Factor at a Time (OFAT) approach, it is unlikely that we would have identified this interaction. Consequently, we would have chosen a riboswitch with reduced robustness and predictability.
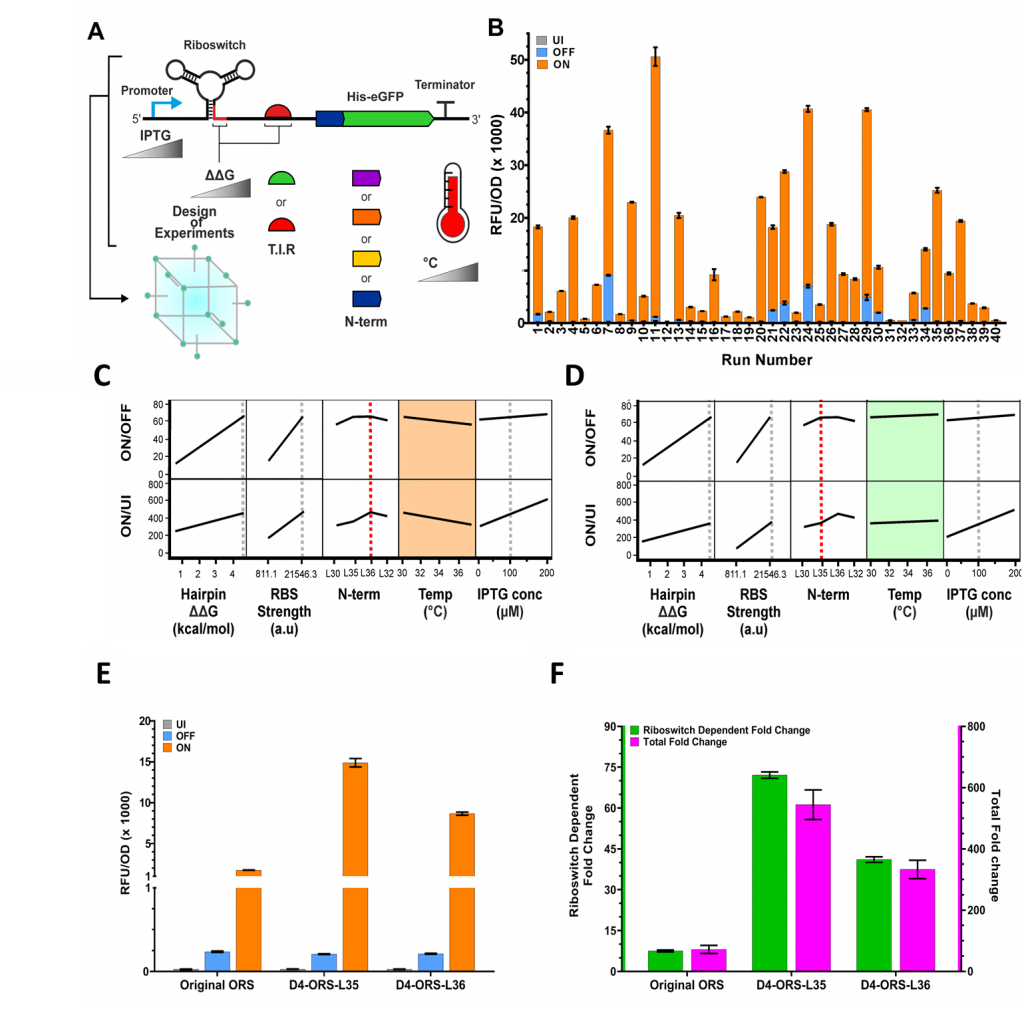
Figure 3. Using Design of Experiments to optimise riboswitch function. (A) Schematic representation of the genetic and environmental factors investigated using Design of Experiments (hairpin ΔΔG, RBS strength, N-terminal synonymous codon variant, temperature and IPTG concentration). (B) Measured uninduced, OFF and ON levels of eGFP expression under each of the experimental conditions as specified by the DoE structure. (C-D) Standard Least Squares modeling of the structure DoE data set predicts an interaction between the N-terminal linker used and temperature. If the model parameters are set to use linker L36 then we see reduced ON/OFF (riboswitch fold change) and ON/UI (total fold change) (C, orange box). However with the model set to linker L35 we do not see this effect (D, green box) suggesting an interaction with incubation temperature. To confirm these model predictions, D4-ORS-L36 and D4-ORS-L35 were both tested. (E) Comparison of UI (grey), OFF (blue), ON (orange), (F) ON/OFF (green) and ON/UI (pink). The original ORS was also included in these comparisons, highlighting the overall improvements achieved by the expression platform engineering and DoE optimisation.
After selecting this highly functional riboswitch, we sought to apply the improved riboswitch. We cloned the optimised orthogonal riboswitch downstream of four endogenous stress promoters (Figure 4). These responsive stress promoter-riboswitch devices allowed us to tune protein expression, in response to both environmental and cellular stress responses, and to modulate expression through PPDA titration.
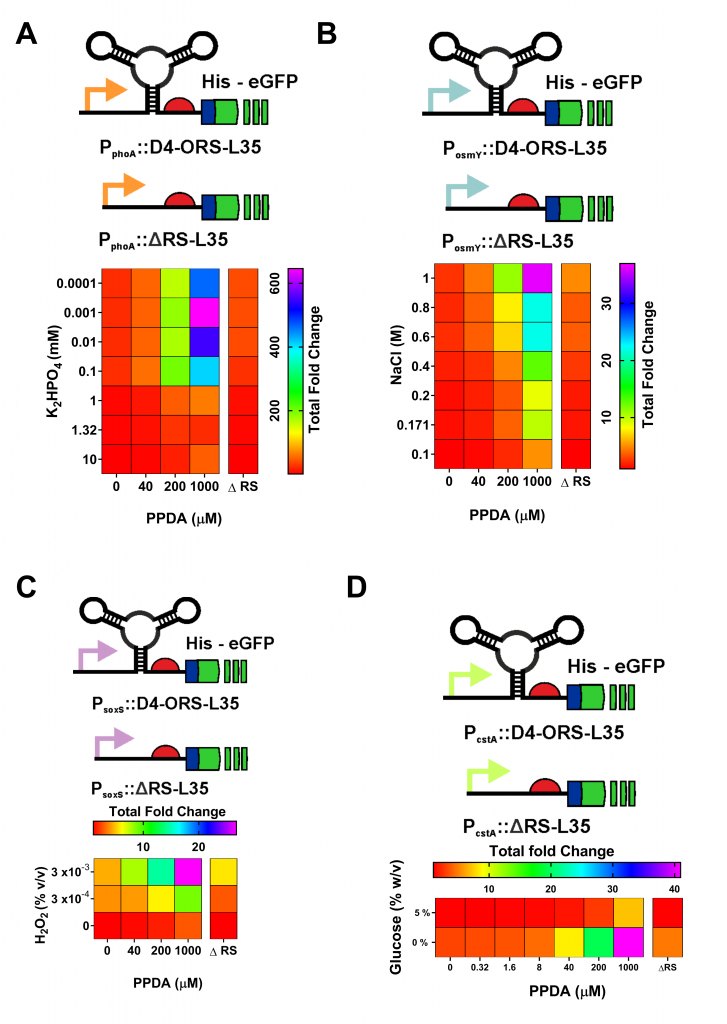
Figure 4. Coupling the D4-ORS riboswitch with endogenous stress response regulation. The D4-ORS-L35 eGFP was placed downstream of 4 E. coli stress response associated promoters. These were: (A) PphoA, (B) PosmY, (C) PsoxS , (D) and PcstA devices, which are activated under phosphate starvation, osmotic stress, redox stress and carbon starvation respectively. These stress responses where selected for their relevance to large scale fermentation. Testing of these devices showed different levels of eGFP expression following differential transcriptional and post-transcriptional induction with PPDA and K2HPO4, NaCl, H2O2, or glucose starvation respectively. ΔRS controls where the ORS has been deleted from each device were included for comparison. Comparison to these controls show how riboswitch integration allows these native promoters to be improved by dramatically increasing the dynamic range of the devices. This improvement is achieved by reducing basal levels of expression.
An Engineering Approach to Increase Riboswitch Performance
To engineer this system, we used an interdisciplinary approach that combines directed evolution, in silico sequence analysis, statistical modeling, and the application of Quality by Design (QbD) principles through Design of Experiments (DoE). As a result, we gained novel insights into riboswitch function, improved understanding of riboswitch context sensitivity, and selected insulator sequences to enable portability of the PPDA riboswitch. The improved riboswitches that we've developed expand the regulatory RNA toolkit, allowing tightly controlled, tuneable regulation of bacterial gene expression. Our methodology serves as a framework for optimising other translation-regulating riboswitches.
Our approach is broadly applicable to the field of synthetic biology. In particular, Design of Experiments is an incredibly useful tool that can be integrated into the Design-Build-Test Cycle. Whether a scientist wishes to screen large numbers of factors, to optimise a genetic device or to improve the robustness of a biological protocol, DoE can enable more efficient, robust, and insightful experimentation. With rising interest in the application of machine learning in biology, structured datasets like those used and generated in this study will become increasingly important. Machine learning usually relies on large, structured datasets. When these are not available, it can be challenging to find datasets that fully cover the full experimental space. However, by designing structured experiments, it is possible to reduce our reliance on large datasets. Ultimately, this reduces data collection costs and allows more scientists, not just those in well-funded labs, to readily apply machine learning to complex biological systems.
References
Ross Kent is an academic who uses Benchling. Interested in learning more?Benchling for Academics