The future of antibody discovery: From target validation to candidate selection
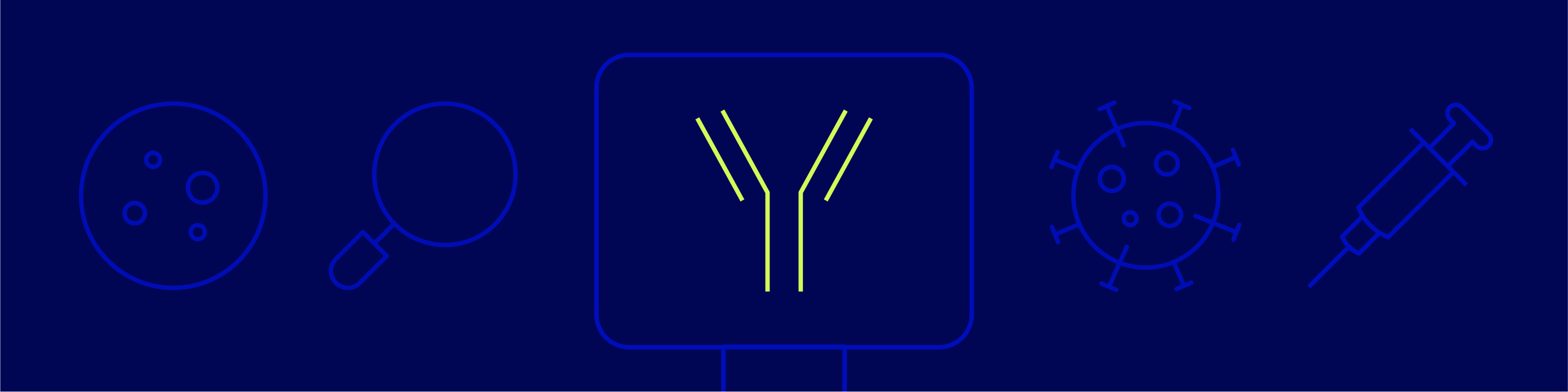
Antibody drug discovery is a diverse topic. In fact, there are multiple ways to dig into it. Sounds daunting? Not for the experts at Benchling. Last month, we came together to discuss some of the most critical steps involved in developing effective antibody-based therapeutics, and how Benchling can support them.
The dynamic duo that introduced some of the most common challenges associated with each step, and the future advancements to tackle them, included:
Sanjay Saraf, Product Manager, Benchling
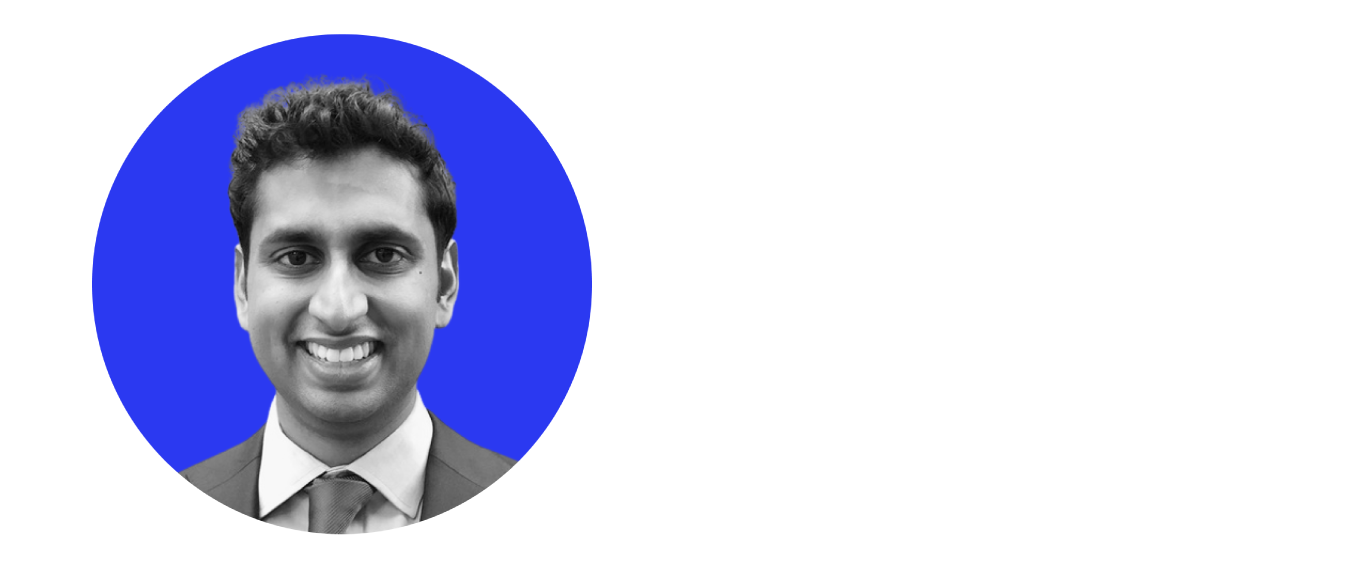
Sanjay Saraf is a Product Manager at Benchling, where he helps biotech companies solve data analysis, automation, and preclinical research problems. He is now focused on Studies, a product which enables scientists to capture accurate, intelligent, and connected in vivo study data. Prior to Benchling, Sanjay led healthcare and biology partnerships at Palantir Technologies, focusing on pharma, government, and clinical organizations.
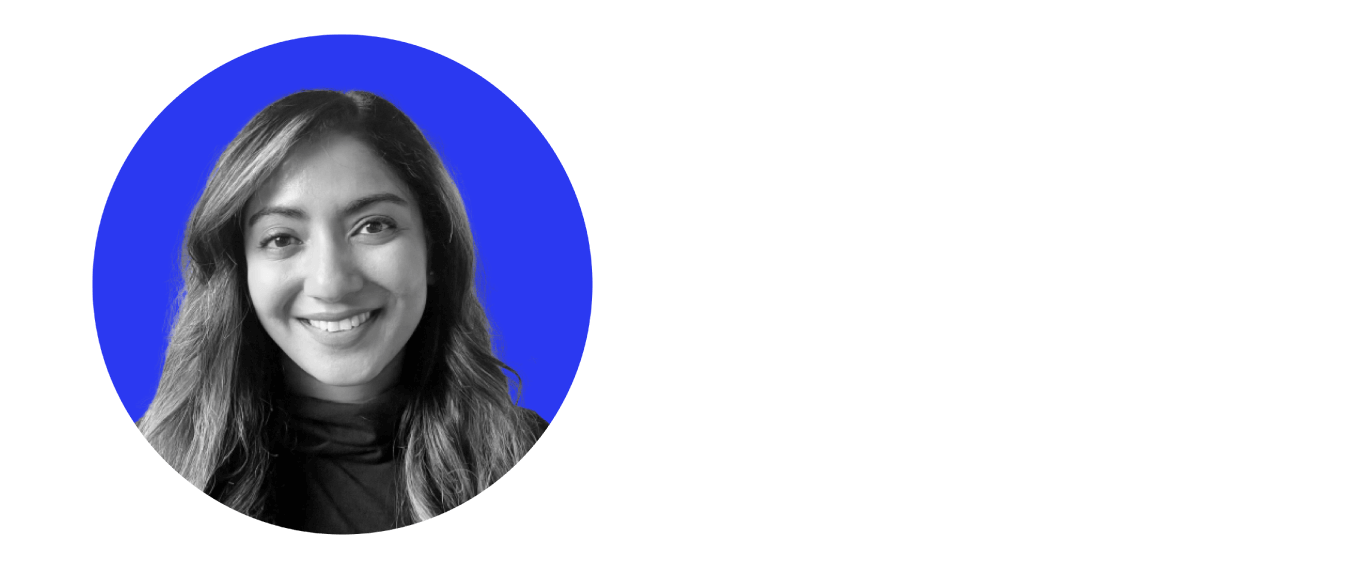
Vega Shah, PhD, Senior Product Marketing Manager, Benchling
Vega is an experienced biologist and former research scientist. In her current role, she helps biotech companies use software to accelerate their research. She previously was a product manager for an electronic lab notebook for biologics and screening. Prior to her industry career she was a scientific researcher at Lawrence Berkeley National Lab, University of Washington, and UC Berkeley.
The hour-long conversation provided an overview of not only the antibody discovery process, but also how Benchling’s Antibody Solution Accelerator can support target identification, lead selection and in vivo safety and efficacy.
The complex world of target identification
Target identification — the first step of identifying and validating specific molecular targets that play a role in disease pathogenesis — is equally complex due to the various different approaches.
The data deluge
Historically, the traditional mechanism for target identification and screening has been paper review. But today, we have much more modern ways of doing things. The problem? These modern methods bring their own set of pain points.
As Sanjay shared, it’s quite common to use retrospective analysis of patient information to try to understand how diseases progress, and why they progress, querying data from a multitude of different places and trying to integrate it together. This plethora of siloed data can cause issues for both qualitative and quantitative insights if not handled and collated effectively.
The future of target ID
Fortunately, there are a host of novel techniques that scientists are beginning to adopt in order to tackle the data deluge.
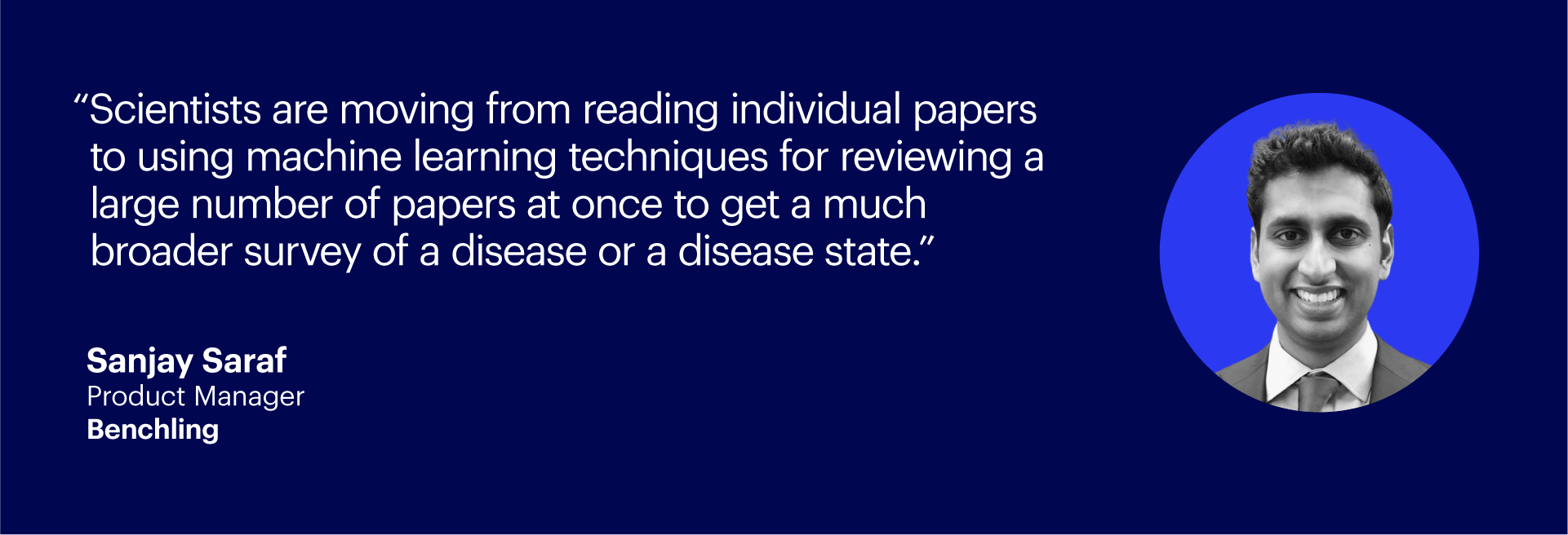
The main goal for understanding a target is to physically look at it, to know where you can attack it to reduce its effect. That structural biology that used to be done manually can now be done today with the assistance of tools, like AlphaFold, that speed up analysis.
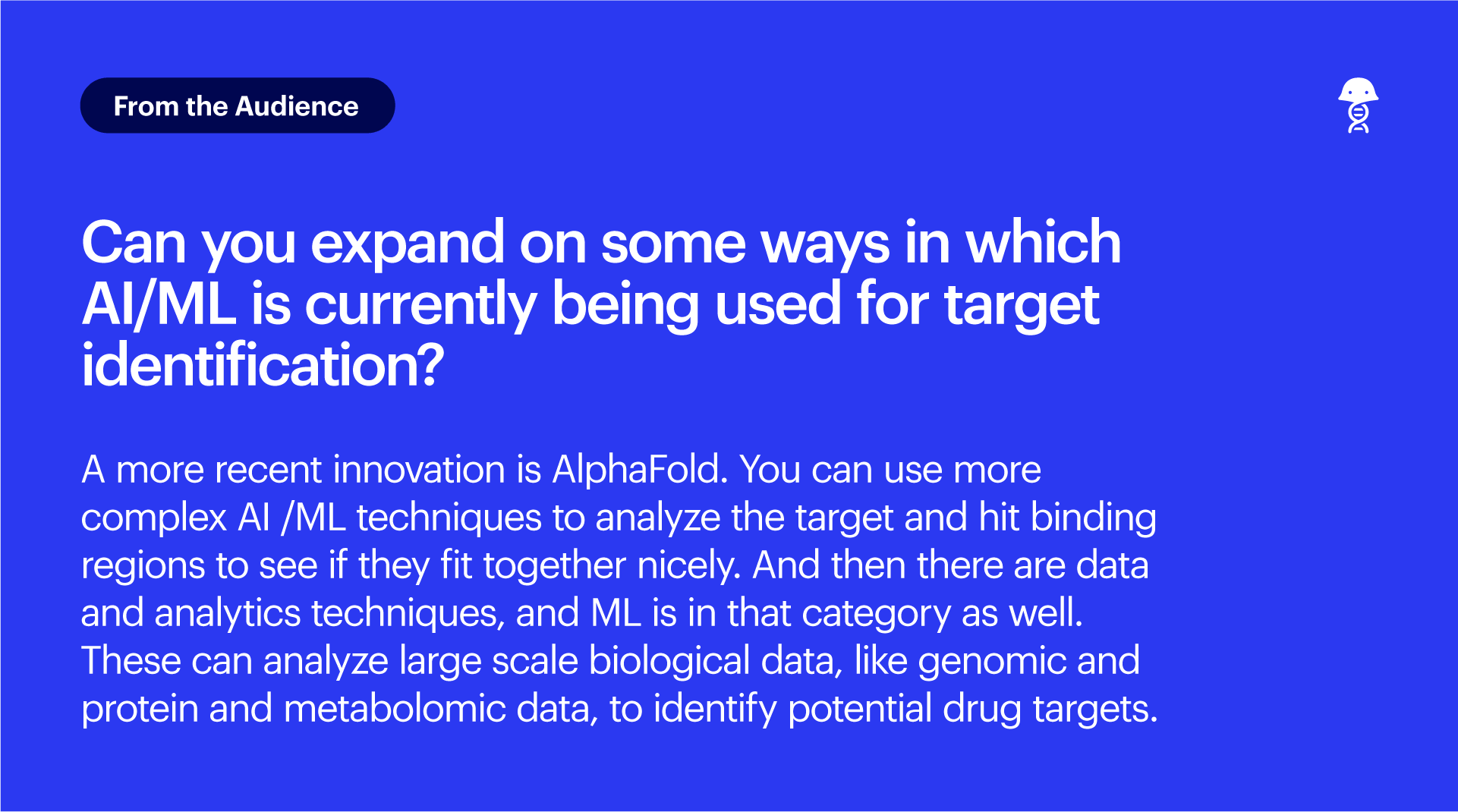
“Throw stuff at the wall and see what sticks”
The next step after target identification is antibody screening. As Vega so accurately calls it, this high throughput screening is, quite literally, like finding a needle in a haystack.
Particularly, with antibody therapeutics, the overall structure of the protein is fairly predictable, but the specific antigen binding sites can vary quite widely. The standard way to generate an ideal antibody is to make a large number of potential antibodies, and throw them at the target to see what binds correctly to the target, and not to other proteins.
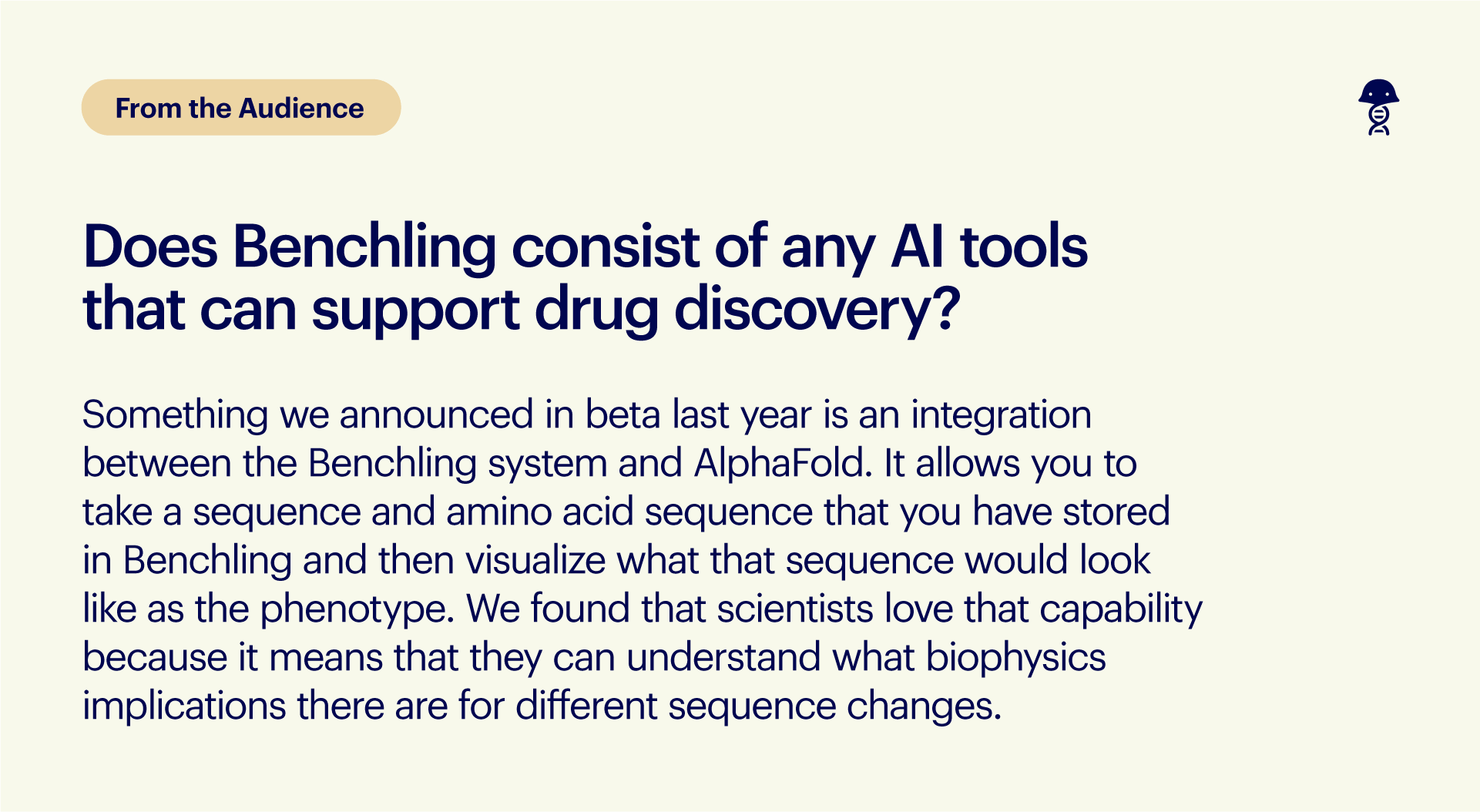
Sanjay walked us through the maturation of techniques for antibody screening. The main mechanism in the past was to inject antigens into an animal for antibody production. More modern techniques can now be used to generate a large number of antibodies that only deviate slightly in their sequences, making it easier to find the ideal protein based on factors such as affinity and specificity.
The chosen one
The process of finding the perfect protein is often known as lead selection and optimization. One of the many things you are trying to do in this process is to produce the perfect candidate, both from a scientific perspective, but also from a practical standpoint of needing certain properties, such as solubility or affinity.
Vega: What are some common issues organizations face in finding the ideal protein?
Sanjay: At this stage, the candidate antibodies are narrowed down to only a few, which are handed off to scientists in a lab to do in vitro assessments of various properties. Scientists will have various data sets, meaning they face the challenge of needing to combine the data together and draw conclusions from it. And so we start to see a siloed data problem, creating challenges in tracking the lineage of a given antibody from its earlier sequence, and not having a source of truth across disparate systems.
Vega: Moving on to where we’re seeing the field going, how are people solving these problems and what are some future advancements?
Sanjay: A lot of siloed data and lineage problems are being solved with web based software that allows for more direct collaboration and standardized data capture.
In terms of future advancements, some of the things that we’re starting to see include far higher throughput, meaning fewer scientists per unit experiment, but many more experiments. We’re also seeing technology such as liquid handlers and automation systems that allow you to run screens and leads across thousands of wells at one time. Also, improvements in our understanding of structural biology, and our ability to use more complex instruments to understand how antibodies are performing, are helping advance this field.
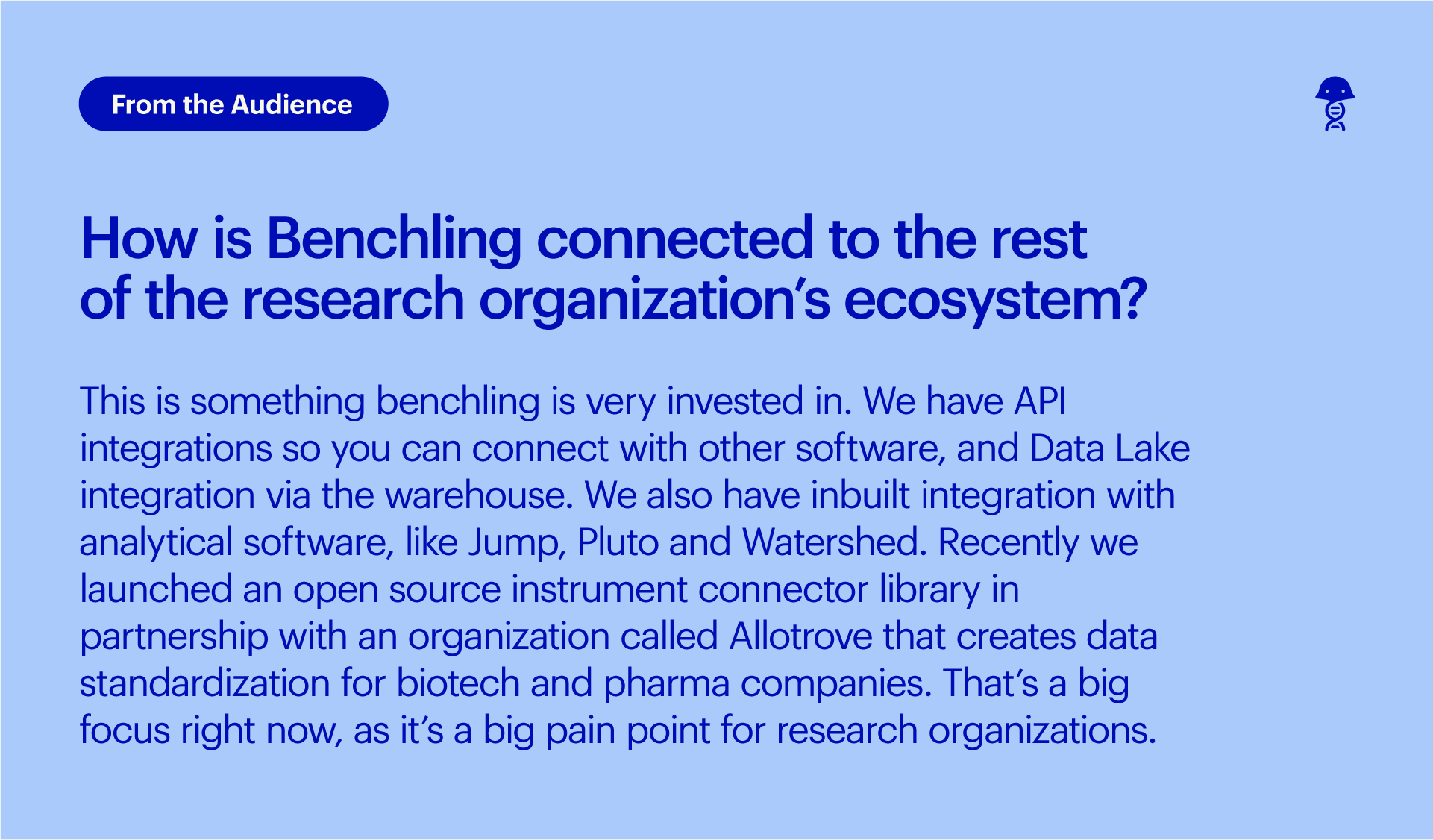
Vega: Right. As technology advances more and more, I’m seeing that it is increasingly more affordable and accessible to have lab robots and software that allows for automation. It’s not just bigger pharmaceutical companies working on this, but also startup and mid sized companies using this in labs. More sophisticated techniques are no longer relegated to large pharma.
Taking it outside the petri dish
The final step in the process serves to de-risk the outcome; what happens in a petri dish is not the same as how something functions in an organism. Often this means that there is an internal handoff from the person who developed the candidate, to the person testing the candidate in the animal.
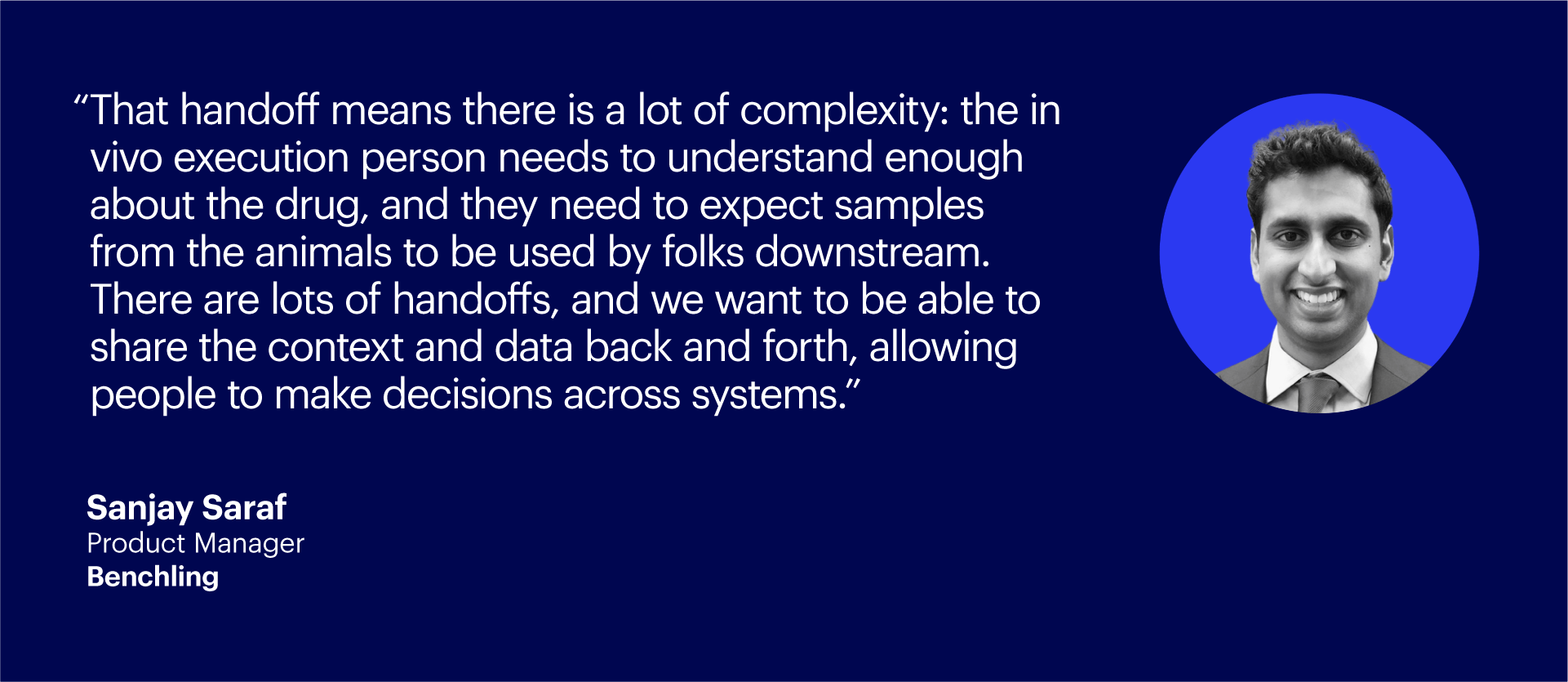
The two then discussed the common role of a contract research organization (CRO) in this final step. There is a cost associated with setting up a vivarium in house, and so pharma organizations, especially smaller ones, will often work with CROs to actually execute a particular individual study.
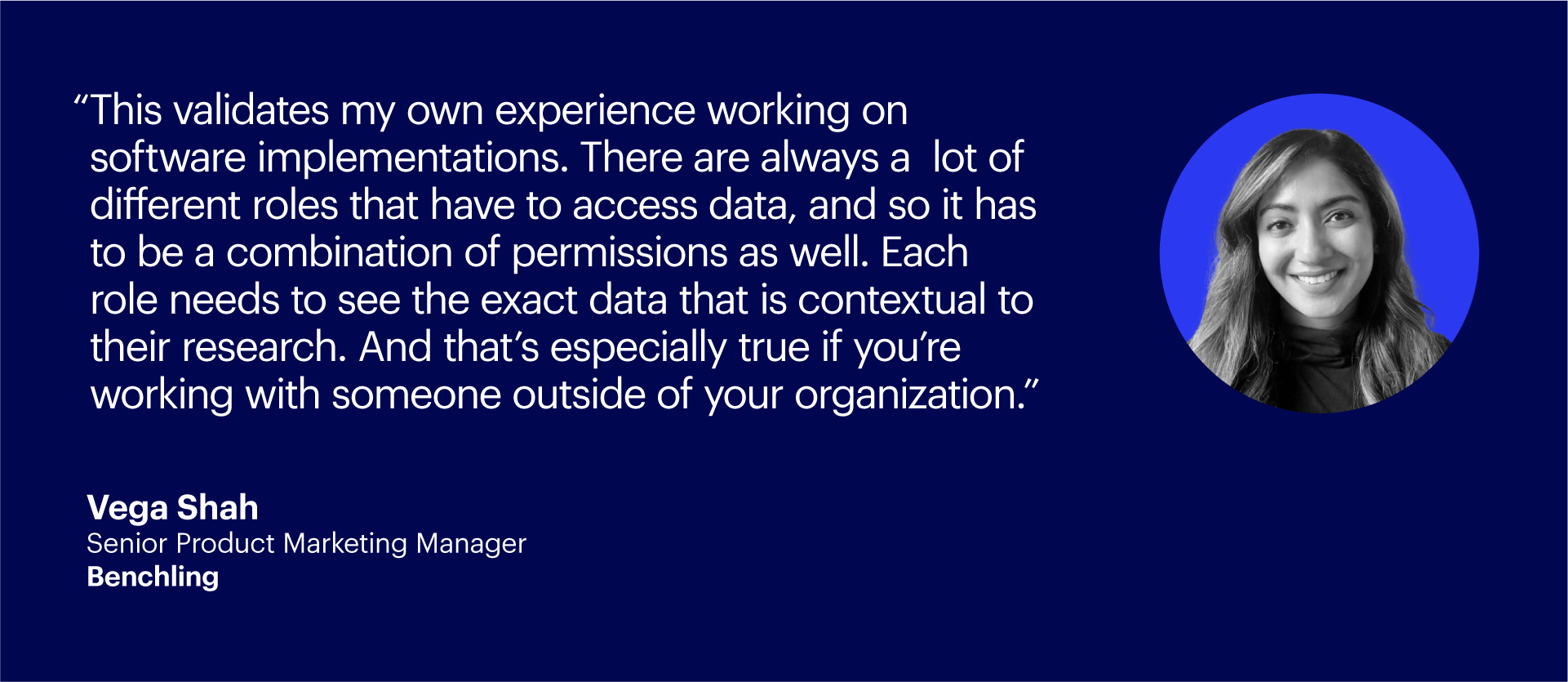
So how are organizations tackling the challenges associated with in vivo? Sanjay shared with us a few places where the field is progressing. In short, it is no longer acceptable to have totally siloed systems between your in vivo and in vitro work. The standard practice is now to integrate the two systems, allowing teams to capture data in vivo, and correlate liver toxicity in animals with gene sequences of antibody therapeutic, for example. Scientists are using more sophisticated imaging and instrumentation for their research as well — such as calipers, scales, and echos — directly tied to their computers to capture data.
Benchling’s antibody and protein research solution
Benchling’s R&D Cloud is a platform to manage R&D data, where almost all teams can pick notebook, registry, and inventory applications to standardize and link data.
With the ability to integrate with other software, scientists can solve for a wide range of needs, from data analysis to data storage. Benchling has an open API that allows organizations to connect to a broader ecosystem. Codeless configuration allows for an advanced level of customization to fit specific needs and modalities, and Benchling’s data warehouse provides storage for large data sets, without the high cost of ownership.
Benchling supports end-to-end antibody research workflows across a range of discovery platforms. Using our extensive knowledge of hundreds of implementations, we’ve created a framework that we see come up very commonly.
Want to learn more about Benchling’s Antibody Solution Accelerator? Let's chat.
Powering breakthroughs for over 1,200 biotechnology companies, from startups to Fortune 500s