4 key insights from pharma leaders on the future of AI
With billions of dollars pouring into the industry, and an intense media spotlight on AI-driven drug discovery, the stakes have never been higher. But AI will fall short if we don’t look at the bigger picture. To ensure success, biopharma companies must take a long-term view on how to adapt their R&D processes and teams to reap the benefits of AI.
The Financial Times gathered industry experts, including Benchling CEO Saji Wickramasekara, Roger Perlmutter (Eikon Therapeutics), Bari Kowal (Regeneron), and Tom Miller (Iambic Therapeutics), to share their predictions for the future of AI — and insights on how pharma will get there. Here are their top takeaways.
Without more data, AI won’t work.
As a former R&D executive at Merck and Amgen, and current CEO of Eikon Therapeutics, Roger Perlmutter is no stranger to defining the cutting edge of biomedical research. At the FT US Pharma & Biotech Summit, he described AI as “the defining tool of our era.”
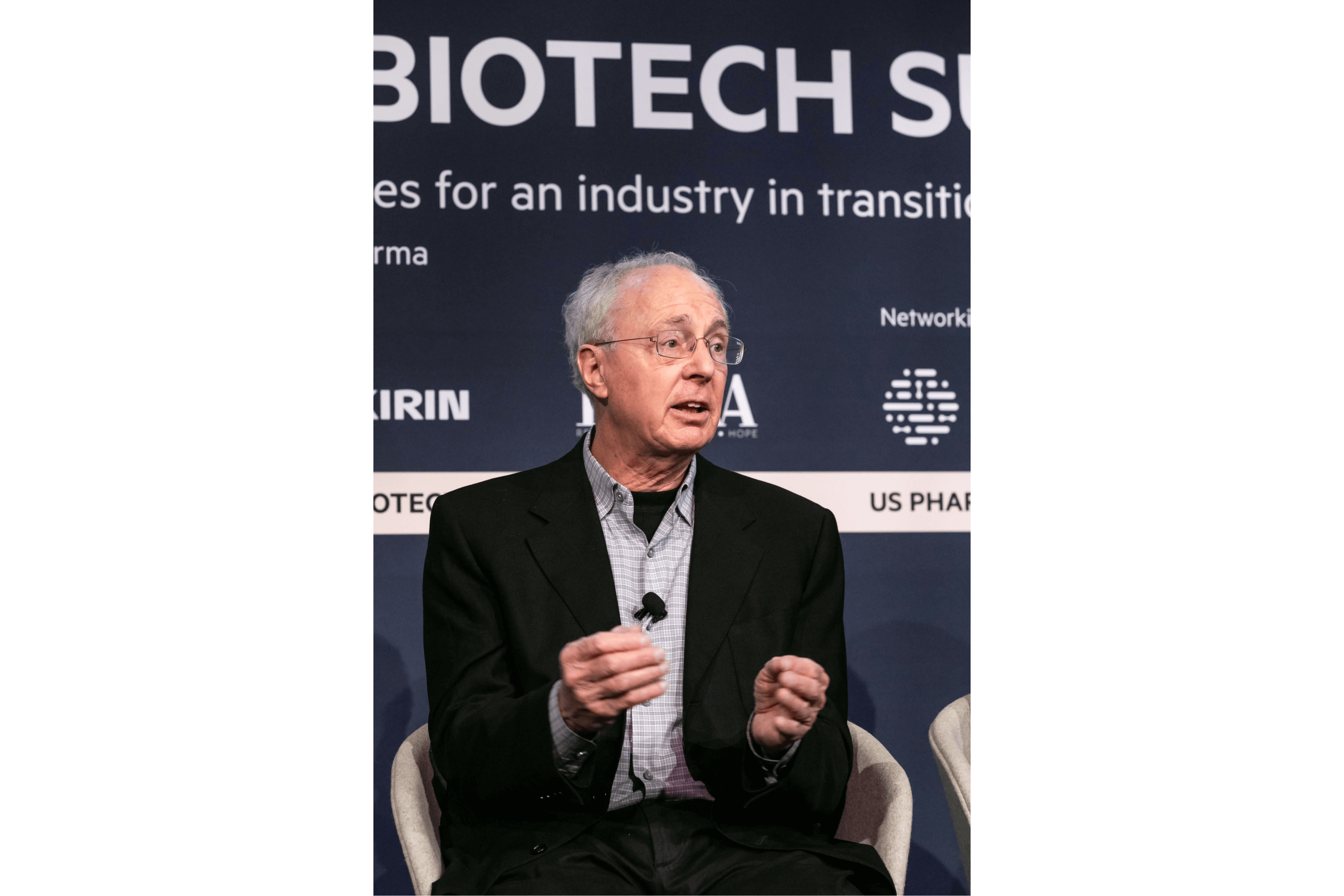
Roger Perlmutter, CEO of Eikon Therapeutics.
Eikon Therapeutics, which uses super-resolution microscopy to observe protein interactions and thereby identify new drug targets, can track thousands of proteins per cell and millions of cells per day. This generates a whopping petabyte of data per day — equivalent to about 250 billion pages of plain text per day.
But with these massive datasets comes the question of how to analyze them. “The reality is that there is no alternative, but to use very high performance computing as a means of trying to examine what the behavior of proteins is inside cells,” said Perlmutter.
Still, applying AI to drug discovery is nowhere near foolproof. Your models will only be as good as the underlying data — and you need a lot of it. “The biggest complaint is always that we don't have enough data,” said Bari Kowal, SVP of Development Operations at Regeneron. “No matter how much data we can produce, whether it's in genomics, where we have large databases of genomic sequencing, or even in the preclinical space — there's just not enough data to really amass the type of learning that you need to be as predictable as we'd like to be.” As all biopharma companies are increasingly discovering, compiling enough data, the right data, is a foundational challenge of implementing AI.
Perlmutter also emphasized how critical it is to build robust models. Given the complexity and scale of interactions even in a single cell, we may never fully “understand” the human body — but we can build models that can, to a measurable degree, make accurate predictions.
“I think we’re at the precipice of figuring out how to go further down that road, but we’re not there yet,” said Kowal.
So how do we get there? “With respect to data, quantity has a quality all its own,” said Perlmutter. “And a large quantity of data enables you to do things that otherwise are unimaginable.”
Data must be captured in a structured environment.
When asked to identify the major limiting factor for AI today, Perlmutter pointed to the challenge of siloed, inaccessible data. An enormous amount of data already exists — but teams must be able to make sense of it five, even ten years later. All too often, the data are useless without additional context from a former employee, and might require an outdated machine that no longer exists, to even read it.
What’s the solution? “Ensure that every piece of data is captured in a structured environment,” said Perlmutter.
But, Benchling’s CEO observed, this can be especially difficult for larger, more mature companies, as opposed to those built specifically with AI in mind.
“For some of the larger organizations, if you look at their research ecosystem, you’re dealing with hundreds of different applications,” said Wickramasekara. “Being able to tie all those together and centralize the data, so you can run them through models, is actually quite challenging.”
As AI continues to improve and become ever more ubiquitous, so too will the need for a robust data infrastructure — one that captures standardized, centralized data to input directly into those models.
AI will never fully replace scientists.
While the power of AI lies in its ability to ingest massive datasets and arrive at insights that no human ever could, failing to appreciate the criticality of the scientist in this loop is a mistake.
As Kowal puts it, “I don’t know that it will replace, ultimately, the science.”
Bari Kowal, SVP of Development Operations at Regeneron.
“As we’ve seen from ChatGPT and other large language models, human reinforcement learning is an essential part of any training of a large model,” said Tom Miller, CEO and co-founder of Iambic Therapeutics.
At Iambic Therapeutics, they bring AI-generated molecular designs back to the lab to generate new experimental data, which then gets fed back into their models for reinforcement learning — all at the pace of thousands of molecules each week. Iambic, which uses AI to accelerate drug discovery, recently dosed their first human patient in a phase I clinical study of a new cancer therapy.
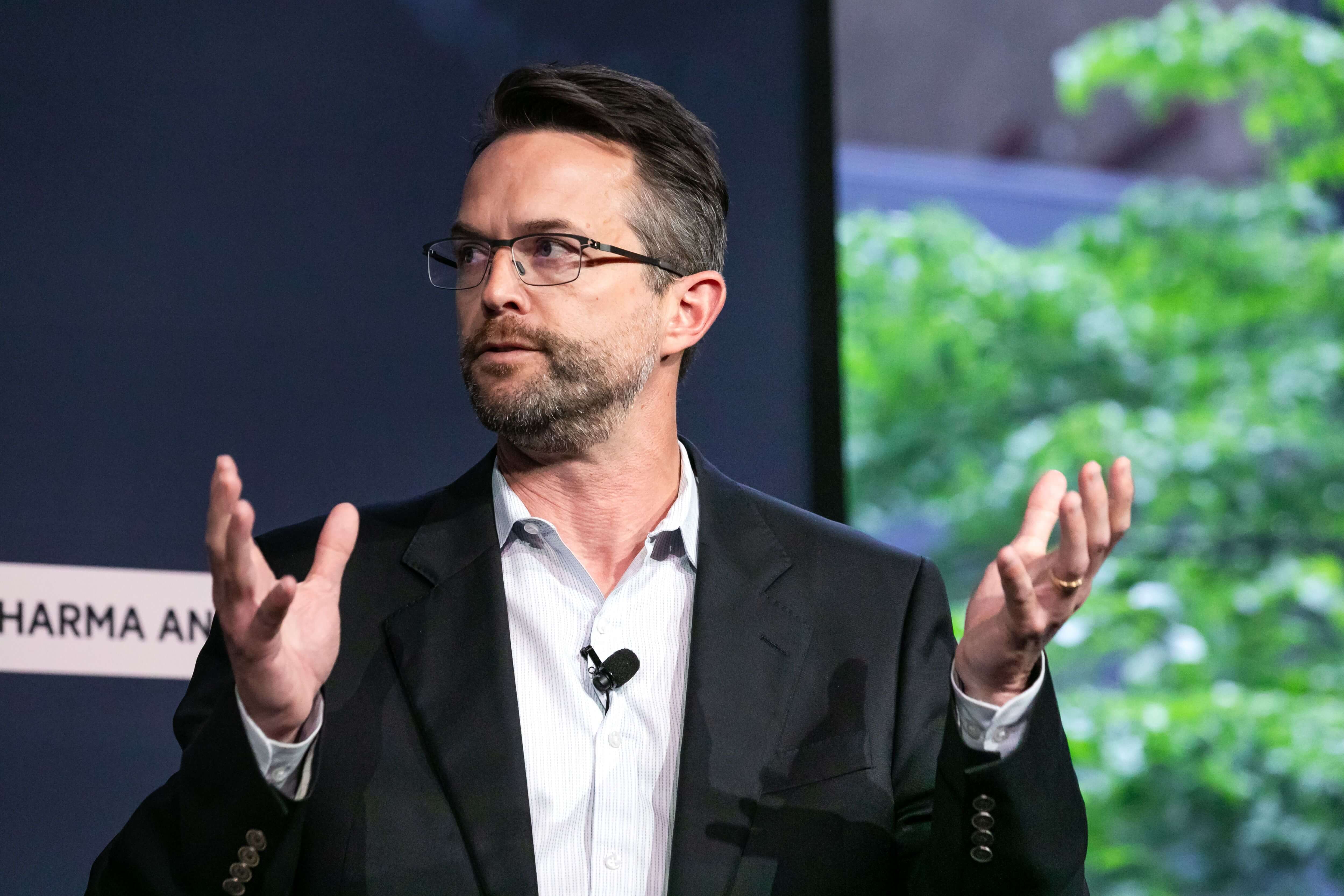
Tom Miller, CEO and co-founder of Iambic Therapeutics.
“Clinical readouts take a long time. Animal studies take a long time,” said Miller. “So finding ways to gather that reinforcement learning to make the models better is something that requires purposeful strategy.”
It becomes critical to design a robust interface between AI predictions and the resulting experimental data needed to train your models. Iterating and continuing to optimize your model is the best way to improve predictions, whether you’re developing new therapeutics, identifying drug targets, or doing something else entirely.
“The software alone will never solve the problem of that search,” said Miller.
AI will accelerate R&D beyond drug discovery.
At Regeneron, there are 400 data scientists — but they’re not necessarily working on discovering new therapeutic targets. Much of the hype around AI has centered on drug discovery, but Kowal’s excitement lies elsewhere: “To me, one of the magic bullets of AI is operational efficiency, so it doesn’t take a decade to get from discovery to a marketed product.”
For Regeneron, this means using AI to take a closer look at other R&D processes, from identifying patients and clinical trial locations, to managing image and data quality, and even making document submission more efficient.
“There’s an insane amount of drudgery in being a scientist,” said Benchling CEO Wickramasekara. “I hope that great technology will help scientists be more effective, and get rid of the difficult administrative tasks that get in the way of doing actual science.”
What does this all mean for the future of AI?
New applications for AI, from the mundane to the fantastical, are emerging every day. AI is fast becoming the tool that helps accelerate every stage of the R&D lifecycle — getting us to world-changing breakthroughs, faster. But it’ll take radical changes to our R&D processes and teams to get there.
“It draws into sharp focus that the real value creation is not the AI algorithm,” said Miller. “It’s the degree to which you can make better medicines and actually deliver those to patients.”
Download our guide on AI for biotech
Read more on getting started with LLMs in our guide to generative AI for biotech.
Powering breakthroughs for over 1,200 biotechnology companies, from startups to Fortune 500s