The State of Artificial Intelligence in Agriculture Research
Big data and artificial intelligence are rapidly infiltrating many industries, and plant biotechnology is no exception. From the lab, to the greenhouse, to the field, and back to the lab, the role played by artificial intelligence in agriculture is growing.
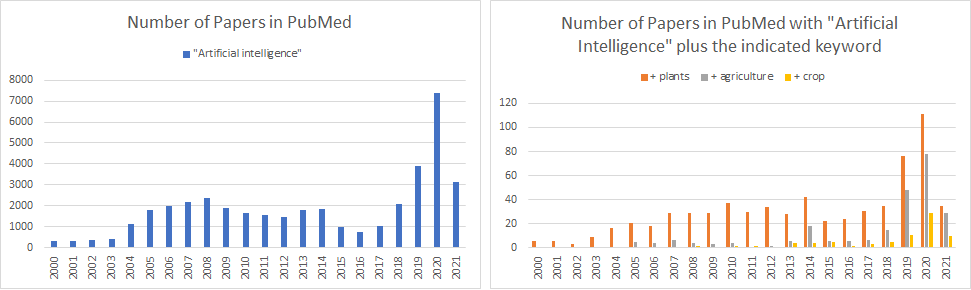
The number of papers featuring artificial intelligence – and artificial intelligence specifically in agriculture research – has increased dramatically in recent years.
Benchling accelerates R&D at dozens of agricultural biotechnology companies, streamlining and unifying their digital lab operations so that data can efficiently flow into artificial intelligence models, and so that insights can flow back into the lab. We’ve assembled a white paper overview of recent literature on how organizations are using artificial intelligence in agriculture, “How Artificial Intelligence Is Advancing Plant Biology.”
Read on for highlights from the white paper.
Artificial intelligence in agriculture: Applications
As in other industries, artificial intelligence is being used in agricultural R&D in two main ways: to automate labor-intensive processes and to uncover insights from complex, multi-parameter data. By accomplishing these two general goals, artificial intelligence is starting to accelerate crop improvement, speed disease detection, quickly optimize in vitro plant tissue culture, and tease apart the tightly intertwined interactions between genetic and environmental forces that drive plant growth, resilience, and crop yield.
The literature makes it clear that we’re still in the early days of artificial intelligence in agriculture, and that there’s a great deal of advancement yet to come. While the intersection of inexpensive next generation sequencing and more powerful computer processors has enabled genomic selection and genomic prediction using linear models, the addition of artificial neural networks and deep learning promises to increase prediction accuracy in the coming years.
In addition, advances in drone, camera, and multispectral imaging technologies combined with detailed, hyperlocal environmental tracking is making it easier to build detailed data sets on the impacts of environment on plant biology in the field. These data sets can feed into genomic selection models, adding a critical layer of environmental information that is missing from many current approaches.
It’s worth noting that in our overview of the literature, we focus on agricultural R&D rather than precision agriculture, which relies on drones, robots, and sensors to automate as much of the soil preparation, planting, growing, and harvesting process as possible. While there’s clearly a lot of efficiency and productivity to be gained through precision agriculture, these are primarily engineering challenges, and Benchling’s focus is on enabling life science R&D. With Benchling, AgBio companies can maintain efficient digital lab operations that streamline the process of feeding data into artificial intelligence models and ensuring that the insights generated become part of the relevant digital records.
Artificial intelligence in agriculture: Algorithms
The publications we highlight in this white paper use a variety of artificial intelligence algorithms and approaches. While each algorithm is best suited to a specific type of problem, we found that many of the most successful machine learning and deep learning approaches used a stacked-ensemble method, which combines multiple machine learning algorithms.
In addition, free tool kits such as Ilastik [1] have become more prevalent in recent years. Such tool kits are designed to help domain specialists like plant biology researchers more easily take advantage of the power of machine learning for activities such as object detection and classification. As artificial intelligence matures as a field, AI capabilities are increasingly being packaged into platforms that are accessible to non-specialists.
Artificial intelligence in agriculture: It’s the future
With the potential to help researchers learn more and learn more quickly, artificial intelligence is increasingly being used to help AgBio companies breed better plants, optimize growth, and understand the complex interplay between genetics and the environment. Benchling is proud to be part of the process for a number of AgBio companies, helping them build efficient and unified digital operations that support artificial intelligence-driven insights.
Learn how Benchling can accelerate your agriculture R&D workflows.
References
1. C. Sommer, C. Straehle, U. Köthe and F. A. Hamprecht, "Ilastik: Interactive learning and segmentation toolkit," 2011 IEEE International Symposium on Biomedical Imaging: From Nano to Macro, Chicago, IL, USA, 2011, pp. 230-233, doi: 10.1109/ISBI.2011.5872394.