The power of software-led antibody drug discovery
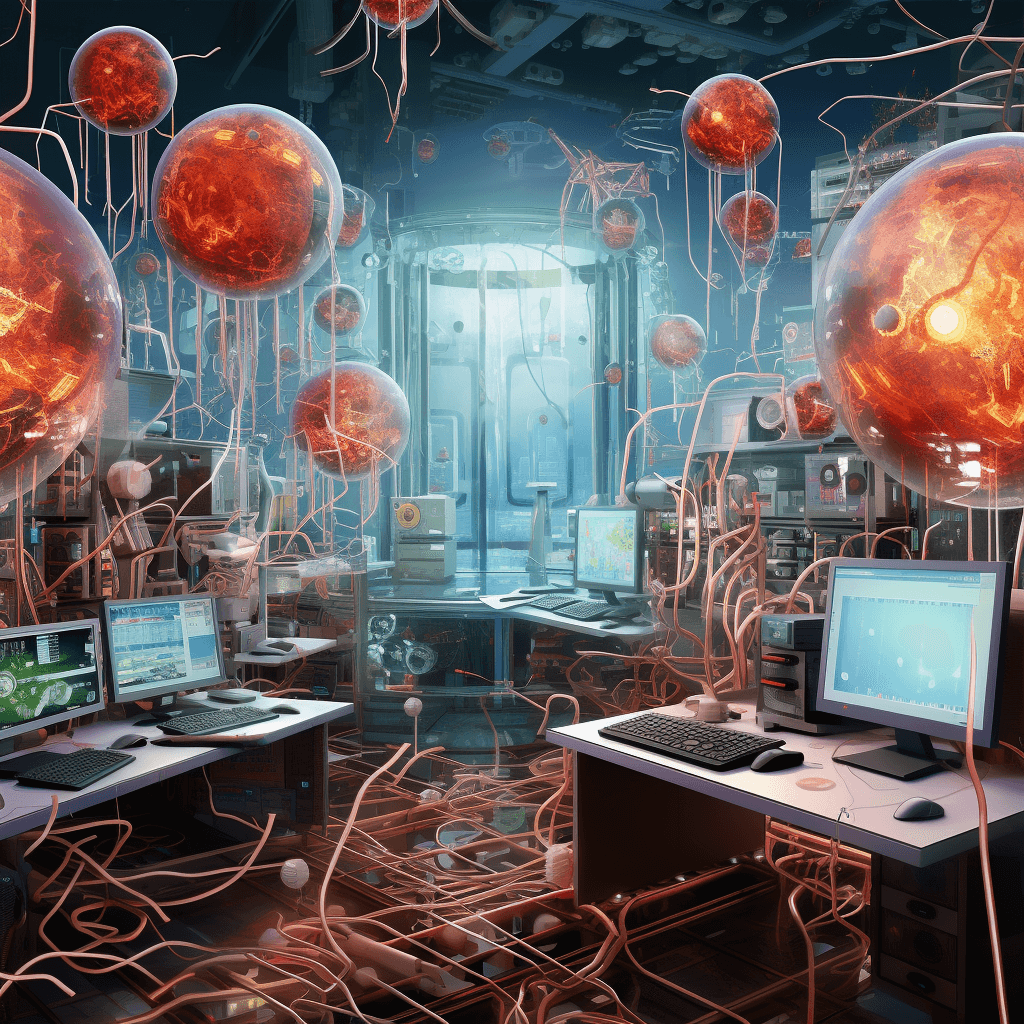
In biotech and pharma, antibodies have historically been powerful therapeutic agents, capable of targeting specific disease pathways and enhancing the body's immune response. The discovery and development of antibodies has relied on labor-intensive and time-consuming experimental methods for target identification, screening, lead optimization, and preclinical research.
However, a paradigm shift is occurring with the rise of software-led antibody drug discovery. Artificial intelligence and machine learning can accelerate the research process, leading to faster and more efficient identification of antibody candidates. In this article, we explore the innovative software-led approaches revolutionizing antibody drug discovery, highlighting some remarkable examples of their application.
Target identification
One of the crucial steps in antibody drug discovery is identifying appropriate disease targets. AI/ML algorithms have demonstrated immense potential in sifting through vast amounts of published literature and genomic and proteomic data to identify potential targets. By analyzing patient data, genetic databases, and previous research information, software-led approaches can detect patterns, predict protein structures, and identify ‘attack’ worthy targets in the disease-causing pathway.
Noteworthy examples of AI/ML-driven target identification can be found in companies like Insitro and Recursion Pharmaceuticals. These companies have harnessed the potential of AI algorithms to delve into the complexities of diseases such as cancer, liver disease and neurodegenerative disorders. Through this approach, they have successfully uncovered novel disease targets that were previously challenging to identify using traditional methods.
For instance, Insitro uses AI-based techniques to analyze large-scale genomic and clinical data to identify promising targets for liver diseases and create innovative therapies. Recursion Pharmaceuticals, on the other hand, uses ML algorithms to analyze biological images, thereby identifying potential disease targets and screening thousands of compounds for drug development to treat conditions like rare genetic diseases.
Antibody design
Once a target has been identified, the next step is designing an antibody that can specifically bind to and modify the target’s activity. This process traditionally involved experimental screening of large antibody libraries. However, software-driven approaches are transforming this process by employing computational modeling and simulations. By analyzing the target's structure and dynamics, these methods can predict antibody-antigen interactions, identify potential binding sites, and help design optimized antibody variants with higher affinity and specificity. Companies like Arzeda and Absci are using AI-driven platforms to design novel antibodies with improved therapeutic properties.
Researchers at the University of California San Diego School of Medicine recently made a breakthrough by devising an innovative AI method for uncovering high-affinity antibody drugs. The study published in Nature Communications, showcases the successful implementation of computational antibody design. Through their approach, the scientists identified a novel antibody that demonstrates a 17-fold increase in binding affinity to a major cancer target when compared to an existing antibody drug. This groundbreaking pipeline holds the potential to revolutionize the drug discovery process, significantly expediting the identification of novel drugs to combat diseases such as cancer, COVID-19, and rheumatoid arthritis.
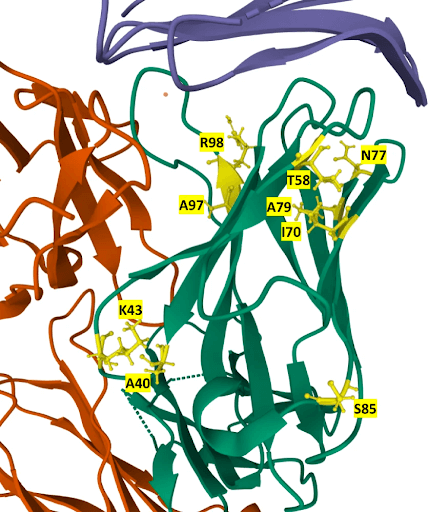
Location of mutations in the 21 mutants in the structure of the Atezolizumab heavy chain. Mutated residues are labeled and colored in yellow in the heavy chain (green). Source.
High-throughput screening and optimization
Antibody screening and optimization are crucial steps in the development of therapeutic antibodies or monoclonal antibodies (mAbs). These antibodies are engineered to target specific antigens, such as proteins or cells, and are used to treat cancer, autoimmune disorders, and infectious diseases. Software-based approaches have revolutionized the screening and optimization of antibody candidates.
Virtual screening techniques, such as molecular docking and molecular dynamics simulations, allow researchers to computationally evaluate antibodies for further experimental validation. By reducing the number of candidates to be tested in the laboratory, these methods significantly expedite the drug discovery process. Moreover, machine learning algorithms can learn from previous experimental data to predict the success of candidate antibodies, allowing researchers to focus on the most promising candidates. Notable examples of companies employing these techniques include Schrödinger and Twist Biosciences.
AlphaFold, a deep learning algorithm developed by DeepMind has recently gained significant attention for its ability to predict the 3D structures of proteins with high accuracy. AlphaFold's predictions can aid in virtual screening of large antibody libraries to identify potential candidates that can bind to target proteins with high specificity and affinity. This approach can significantly accelerate antibody discovery.
The software-led approach will continue to evolve
The integration of software-led approaches such as AI, and ML algorithms in antibody drug discovery have ushered in a new era of rapid and low-labor therapeutic development. By leveraging computational power, these methods enable researchers to identify disease targets, design optimized antibodies, and accelerate development. The examples highlighted in this article represent just a fraction of the remarkable progress being made in the field. As software-led approaches continue to evolve and mature, we can expect a transformative impact on the development of life-saving antibody therapeutics, bringing new hope to patients worldwide.
Read more on antibodies and protein R&D in our blog article Modernize your lab informatics for the next generation of antibody and protein R&D.
References
Eisenstein, M. Machine learning powers biobank-driven drug discovery. Nat Biotechnol 40, 1303–1305 (2022). https://doi.org/10.1038/s41587-022-01457-1
Chandrasekaran SN, Ceulemans H, Boyd JD, Carpenter AE. Image-based profiling for drug discovery: due for a machine-learning upgrade? Nat Rev Drug Discov. 2021 Feb;20(2):145-159. doi: 10.1038/s41573-020-00117-w https://www.ncbi.nlm.nih.gov/pmc/articles/PMC7754181/
Parkinson, J., Hard, R. & Wang, W. The RESP AI model accelerates the identification of tight-binding antibodies. Nat Commun 14, 454 (2023). https://doi.org/10.1038/s41467-023-36028-8
Jumper, J., Evans, R., Pritzel, A. et al. Highly accurate protein structure prediction with AlphaFold. Nature 596, 583–589 (2021). https://doi.org/10.1038/s41586-021-03819-2
Powering breakthroughs for over 1,200 biotechnology companies, from startups to Fortune 500s