The one piece R&D companies are missing in their FAIR data strategy
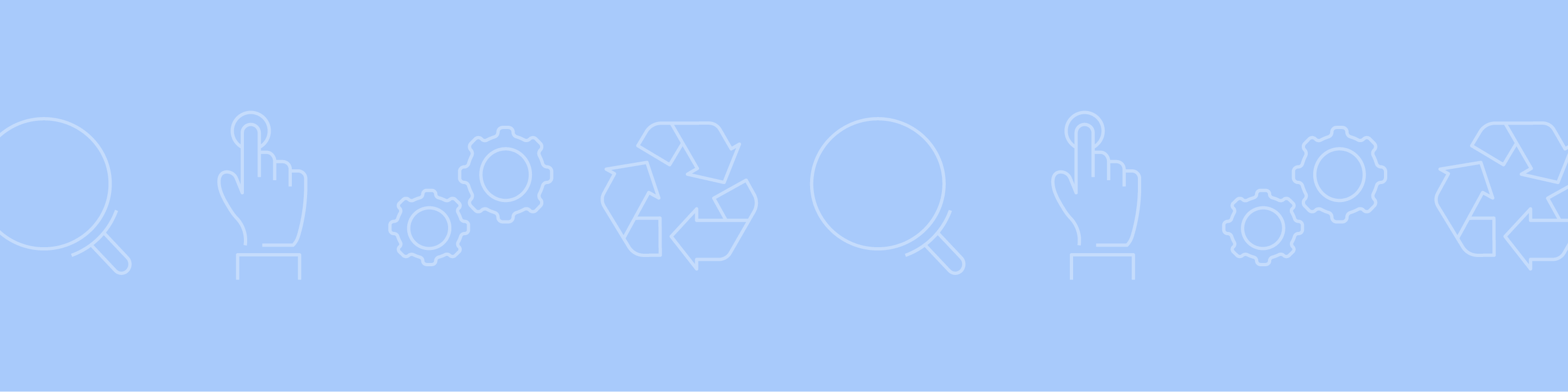
It would be tough to find an R&D leader who isn’t familiar with the FAIR Guiding Principles for scientific data. The principles — which assert that data should be Findable, Accessible, Interoperable, and Reusable — aim to allow people and computers to gain the most value from data, whether it’s public or private, no matter the source it’s coming from. Upholding FAIR data principles has proven to be a significant challenge for R&D companies in particular, who are managing a vast and exponentially-growing range of data types, working with large datasets collected from disconnected sources, and collaborating across many different highly-specialized teams.
There’s no singular “correct” way to achieve FAIR data, given all R&D companies need a specialized approach based on the nature of their specific science. But overall, there’s a critical first step many companies are missing when it comes to implementing a FAIR strategy: FAIR starts with enabling programmatic ownership over data — not just the ability to capture, manage, and engage with their data manually.
While Findability and Accessibility can be measured by interacting with a tool’s human interface, the Interoperability and Reusability of data is dictated by its ability to be manipulated programmatically — unless we want to return to the days of manually transcribing it.
What does “programmatic ownership” over data mean?
When R&D companies implement a FAIR data strategy, they’re aiming to allow the right individuals across the company to:
Find data — which requires searching by relationships and housing files
Access or export data — which requires viewing and sharing with other teams
Interoperate across datasets — which requires integrating with other software tools and systems
Reuse data — which requires long lived data formats and holistic data retrieval
To achieve these actions, companies often focus their efforts in data standardization and access controls. But companies can’t stop there — those might enable “Finding” and “Accessing” — but “Interoperating” and “Reusing” require more work. Teams need programmatic ownership to actually ensure the Interoperability and Reusability of their data.
Enabling programmatic ownership means creating a structured, automatic method for individuals to access data. To create the environment for individuals, R&D companies need to set up data foundations in an open and flexible way, and choose software that allows this.
Companies need to have four elements in place to truly make this happen: open-source, global standards; a flexible, biology-first data model; an API-first extensible platform; and low and no-code integrations.
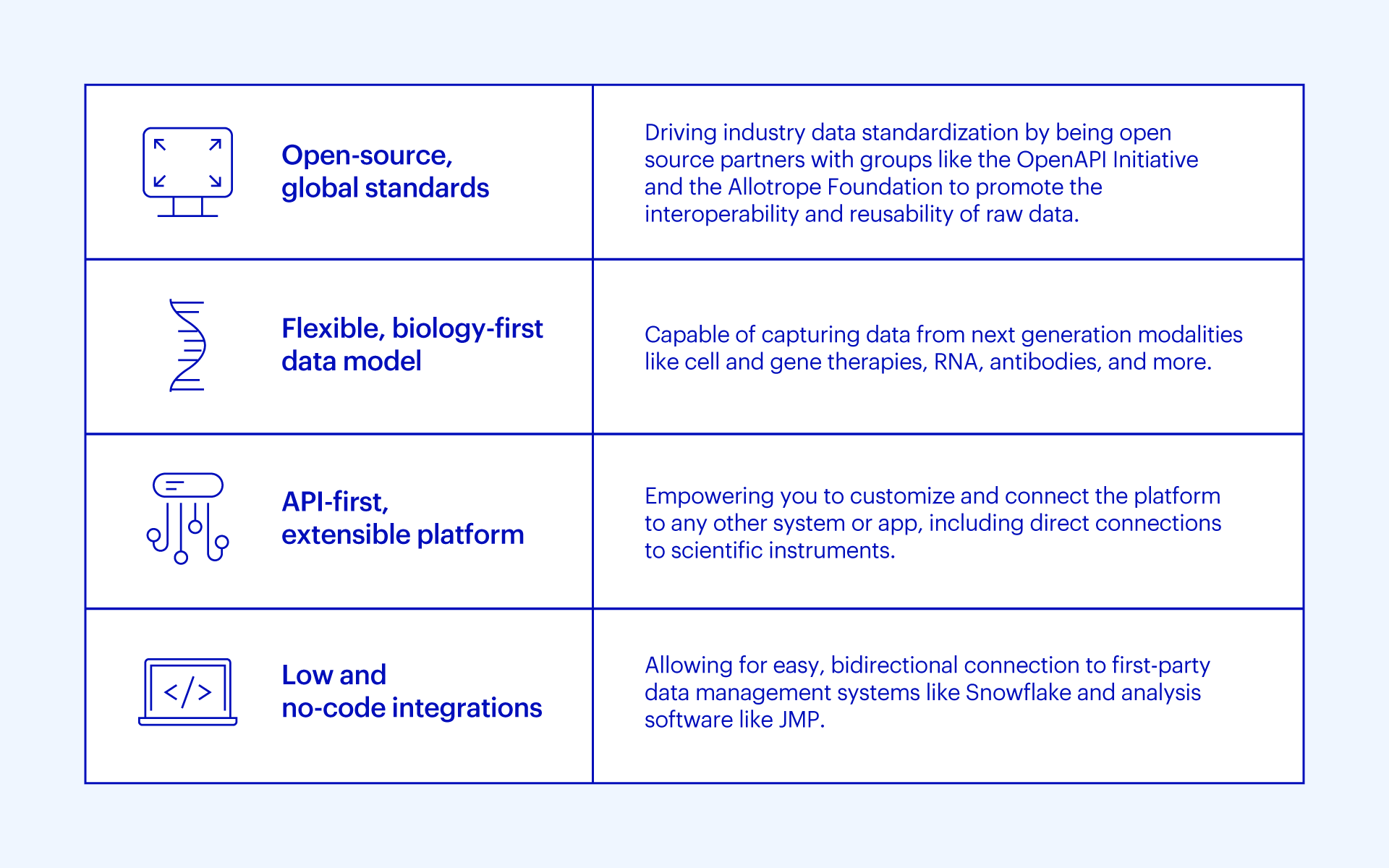
Why move towards FAIR data in R&D?
FAIR data hasn’t been implemented widely across R&D yet because it’s proven to be difficult to implement and manage the change around it. But many agree that a FAIR data strategy for R&D has significant benefits and will become increasingly important as R&D evolves.
In MIT research on FAIRification in pharmaceutical R&D, study participants saw benefits from implementing FAIR data in reusability of study data and cost savings. Study participants noted that “aligning data with FAIR principles would have a positive financial impact on pharmaceutical organizations, as it would enable them to maximize value from their data assets. They explained that the availability of relevant data can prevent the duplication of experiments, which in turn, lowers costs and accelerates timelines across the R&D pipeline.”
They noted that the main costs of “FAIRification” were simply the resources it takes to implement and manage the change resulting from FAIR processes and tools.
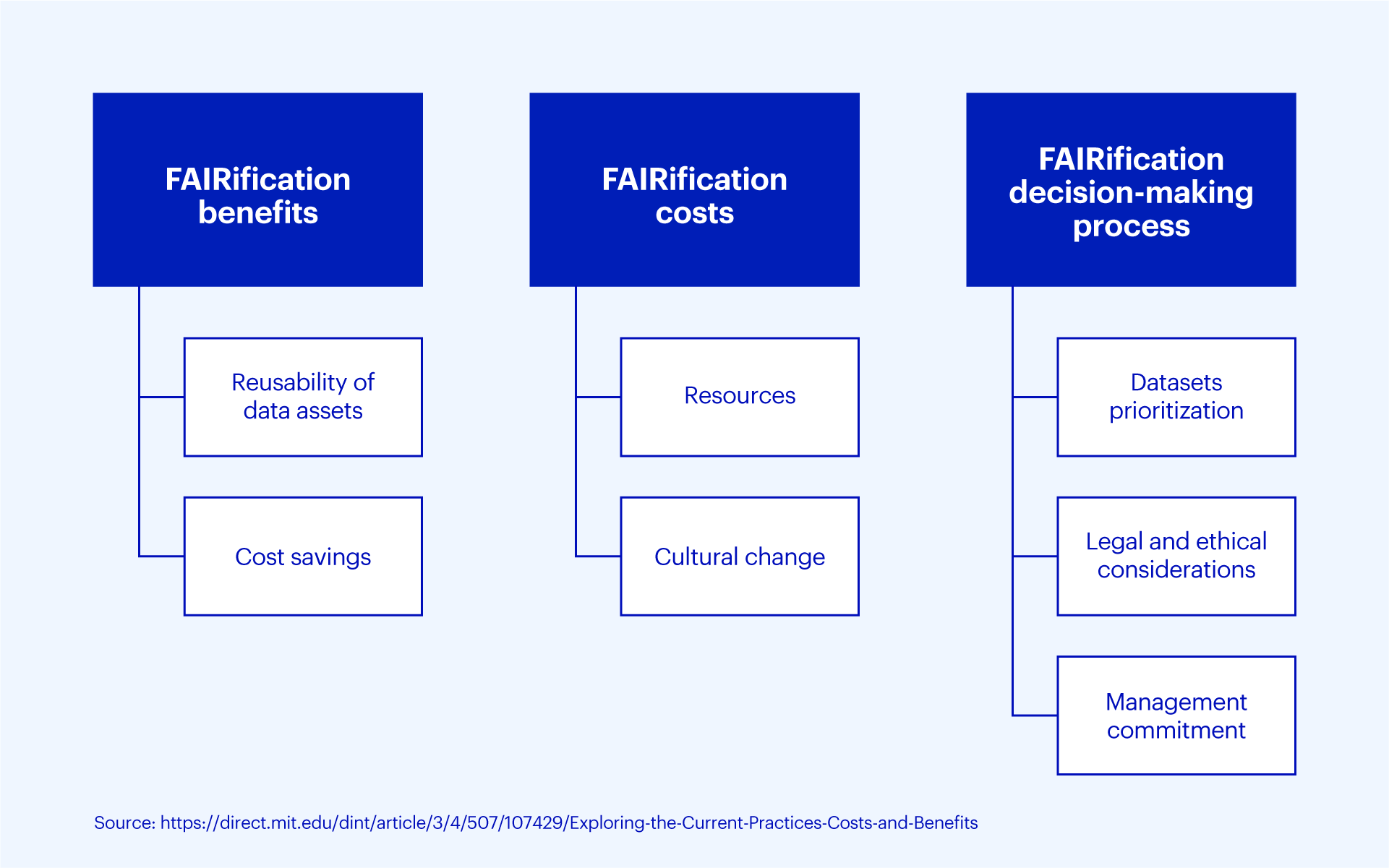
Drug Discovery Today research on implementation of FAIR principles in biopharma R&D noted that short-term benefits were better findability for existing data and quicker access to the data at scale. They also noted that standardized and high-quality data enabled teams with analytics and eventually the ability to bring machine learning into processes. Down the line, these benefits would be measurable in cost savings and faster times to market. The article shares that “Implementing the FAIR principles as a corporate data management strategy results in multiple improvements including the possibility for robotics and process automation through machine readability (of data and metadata), which will enable reuse and scalability…As a consequence, time-to-value will be significantly reduced, productivity will increase, and drug R&D can be accelerated.”
A critical starting point for future innovation
With programmatic ownership comes truly FAIR data — and this sets companies up for the ability to innovate and take advantage of cutting-edge technologies down the line, like AI and ML. According to Benchling’s Software Engineer Janet Matsen and Machine Learning Product Manager Helen Liu-Mayo, “The mistake that we commonly see is that companies jump ahead, and do data science before solving the hard foundational problems of establishing the pipeline and flow of data for analysis. They don’t pause to operationalize the flow of data coming from experimental pipelines for usage by data scientists. As un-sexy as it is, companies need to build their data strategy and systems before benefitting from ML.”
We all know FAIR data is more than just capturing data — but it’s more than being able to manage and engage with it, too. When teams have programmatic ownership over the data they’re working with, that’s when you’re truly operating with a FAIR data strategy.
How to make R&D data your competitive advantage
The pace of scientific discovery and innovation is accelerating. How can biotechnology and life sciences companies get ahead? The key is rethinking the way you capture, manage, and use R&D data.
Powering breakthroughs for over 1,200 biotechnology companies, from startups to Fortune 500s