How to apply FAIR guiding principles to scientific data management
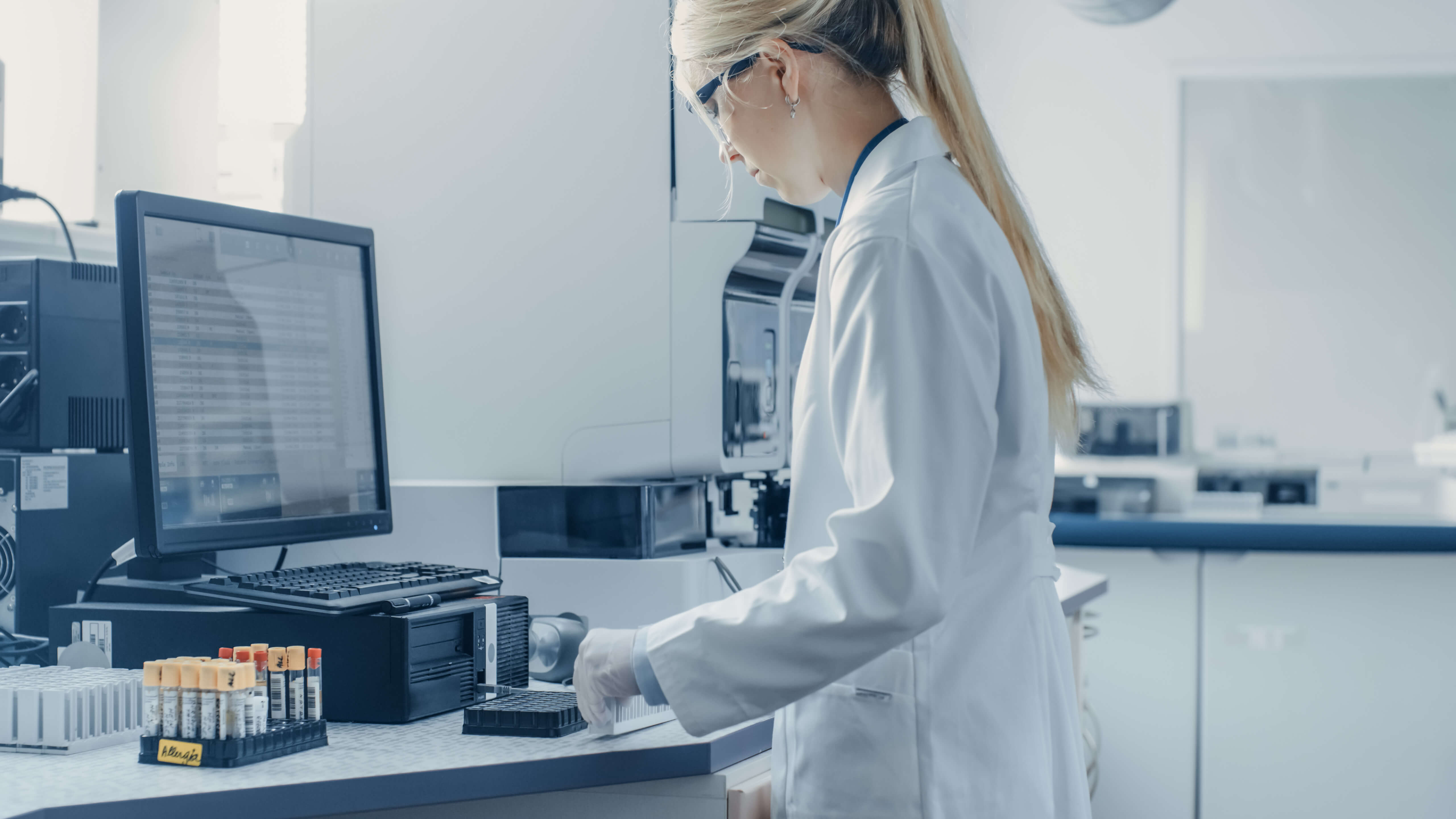
Life science companies sit on a vast amount of data. But large quantities often equate to low quality. Data is often entered manually in an unstructured way. Scientists report only 60% confidence in data accuracy — and half report they struggle to access the insights they need to do their work.
FAIR data principles have become an international guideline for high-quality data management and stewardship, serving as reference points for developing an effective modern data strategy.
While FAIR principles can be applied across many data-producing industries, they're particularly pertinent in life sciences because of the exponentially-growing volume of data being produced in recent years. The National Human Genome Research Institute predicts genomic research will generate between 2 and 40 exabytes of data in the next decade.
Below we’ll share details on the FAIR guiding principles, why they’re so critical for R&D, and how Benchling supports the implementation and upkeep of FAIR across the R&D lifecycle.
What is FAIR data?
In 2016, the ‘FAIR Guiding Principles for scientific data management and stewardship’ were published in Scientific Data. The acronym FAIR states that all digital objects should be “Findable, Accessible, Interoperable, and Reusable”.
The principles emphasize that machines need to be able to act on data, with minimal or no human intervention — because as data volume grows, it’s not only useful but necessary for machines to process large data quantities. FAIR principles state you must be able to:
Find data — which requires searching by relationships and housing files
Access or export data — which requires viewing and sharing with other teams
Interoperate across datasets — which requires integrating with other software tools and systems
Reuse data — which requires long-lived data formats and holistic data retrieval
Let's dig into each in more detail:
Findable
The first order in establishing FAIR principles is to make sure that metadata and data are readily accessible for both humans and computers. Most critical to this step is the assignment of globally unique and persistent identifiers, which removes confusion over the meaning of published data. Data should also include descriptive information about characteristics of the data, such as context or quality, helping to improve searchability. Registering metadata and data in a searchable resource will further protect against data resources going unused.
Accessible
Once the data is found, access, authentication, and authorization come into question. FAIR data should be retrievable from their identifiers, using a standard communication protocol. Because there is a cost to maintaining data online, datasets can often expire over time. Metadata is valuable as a standalone resource, and should persist even when data is no longer available.
Interoperable
Most life science companies use a host of applications and workflows to complete daily tasks. Data is more valuable when integrated with this ecosystem for analysis, storage, and processing, as well as integrated with other data. To facilitate the exchange and interpretation of data, a common understanding of digital objects should be established with a language for knowledge, as well as FAIR-compliant vocabularies. Creating scientific links between datasets further enriches the knowledge of each data resource.
Reusable
Making sure that metadata and data are well-described contributes to the goal of FAIR: optimizing the reuse of data. Including metadata that describes the context under which the data was generated supports the ability of a user to decide if the data is relevant to the specific context.
Why are FAIR principles critical for R&D?
Speed and efficiency have become more vital in such a competitive market, building mounting pressure for research and development teams both big and small. What once took 5-7 years to get a product to market, from discovery to entering the clinic, now takes 3 years based on best-in-class standards. There’s also increasing financial pressure in a highly competitive market — nearly 200 drugs will lose patent exclusivity in just 6 years, making new drug development and innovation a requirement.
As these expectations rise, comprehensive digitalization of R&D is not only helpful, but crucial in winning the race for the next breakthrough. Legacy systems that are not flexible or open slow down progress by limiting knowledge transfer, siloing data, and wasting precious time on data cleanup and management.
FAIR data provides tremendous value for scientific data, specifically by reducing R&D costs, promoting collaboration, enhancing data integrity, and enabling powerful technologies, such as artificial intelligence and machine learning, to solve complex problems.
The challenges of implementing FAIR principles in R&D
In the industry’s current environment of massively growing data, a highly fragmented software landscape, and limited data standards, it’s no surprise organizations are lagging with achieving FAIR data principles.
In Benchling’s 2023 State of Tech in Biopharma Report, a survey of over 300 biopharma executives found that with teams spreading scientific data across 10+ application (20+ at larger companies), there is notably limited progress in achieving organization-wide data interoperability (28%), and data reusability at 30%.
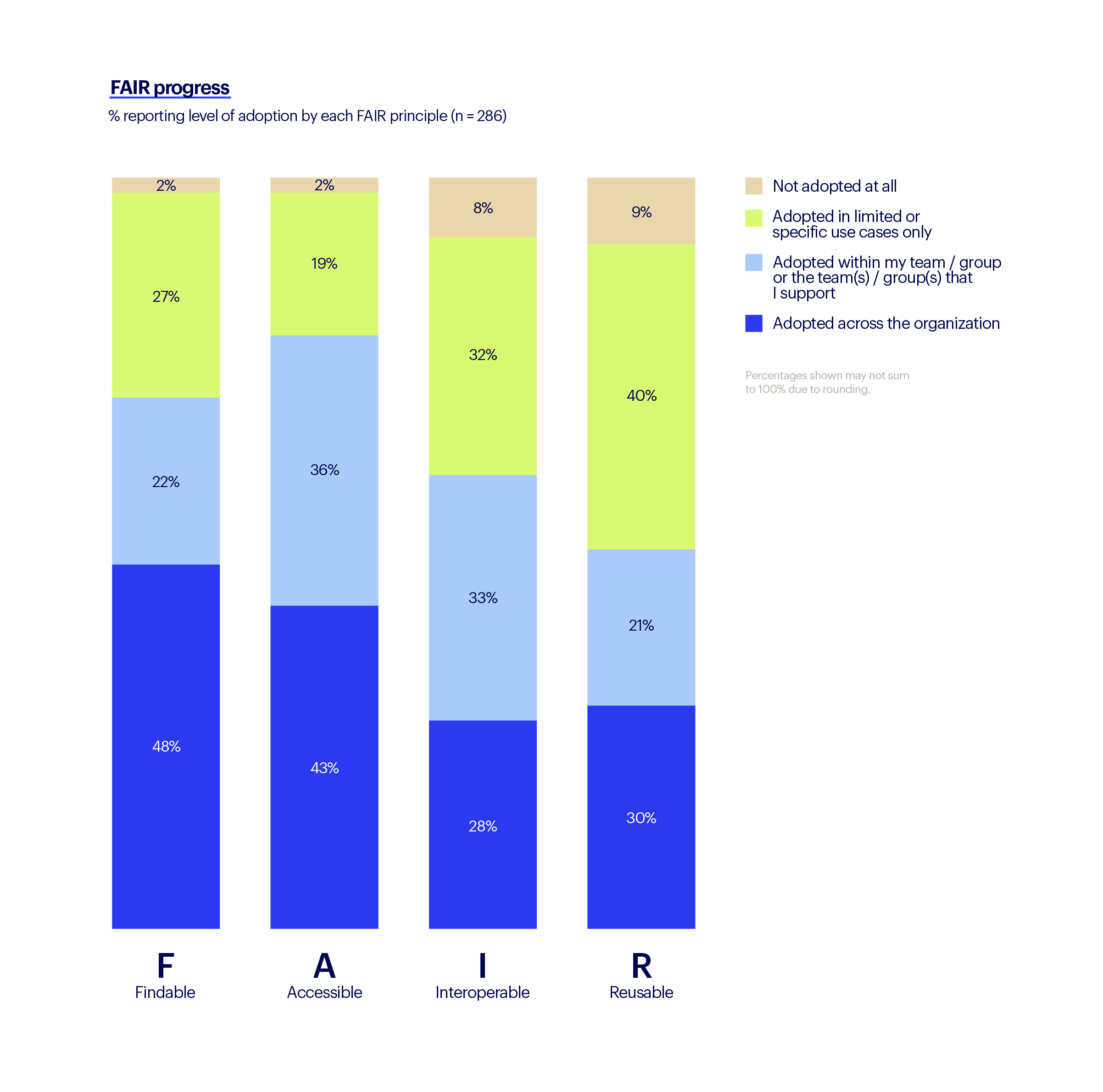
How Benchling supports FAIR principles for R&D
Benchling gives R&D companies a strong digital foundation so teams can capture structured, high-quality data from the start that's easy to find, reuse, and collaborate on.
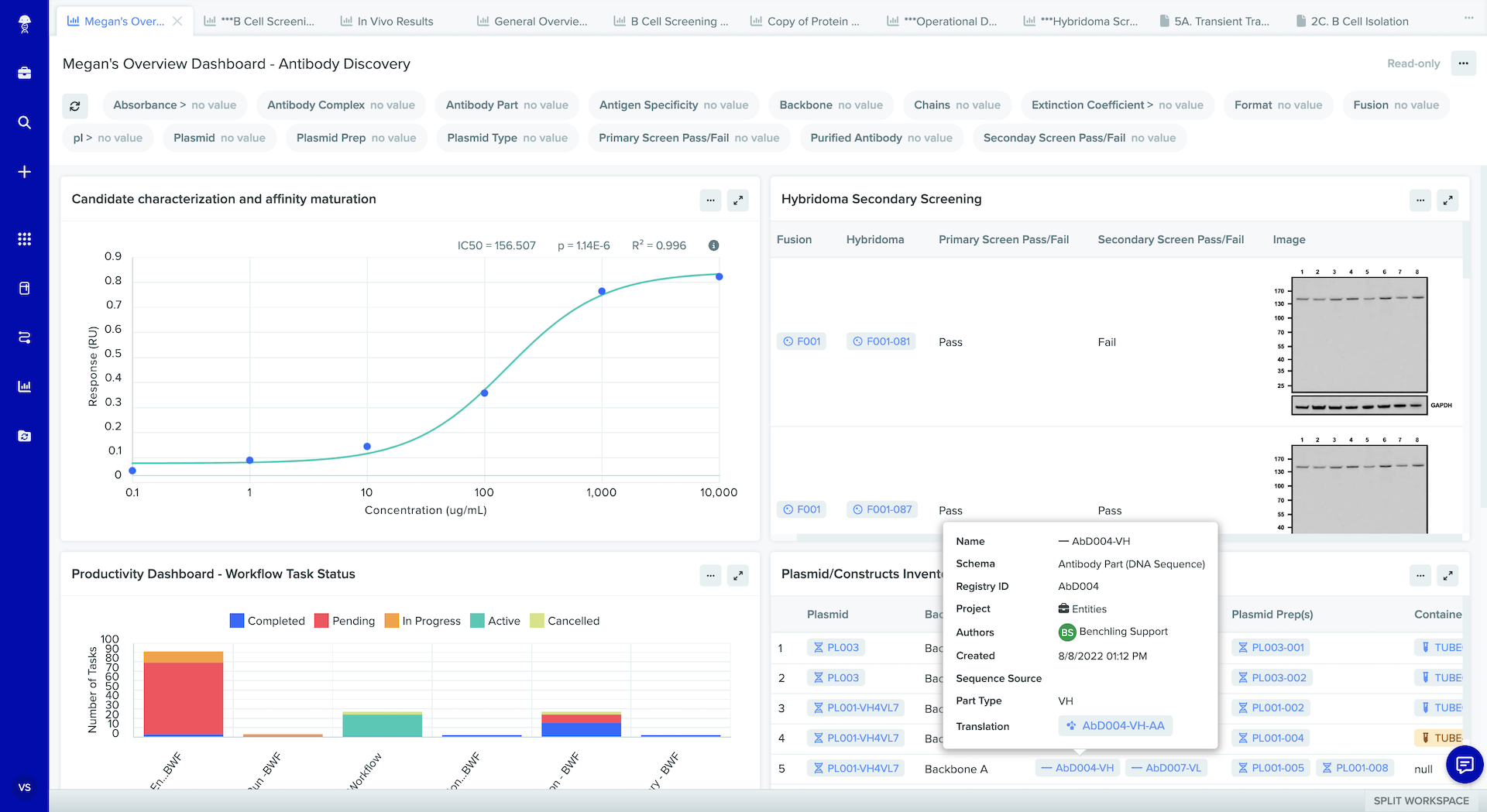
Findability
If a scientist or data scientist is unable to find data, then it’s useless in answering a question or solving a problem. Data must be structured in a way that makes it easily discoverable and query-able by both humans and machines. This requires effective use of unique IDs and rich metadata tags.
With Benchling, each registered entity (such as a cell line or protein) is assigned its own unique ID, with a URL link that serves as the unique and persistent identifier. Every entity can be associated with critical metadata, which not only describes it, but also provides useful context. For example, if the entity is an antibody, then the metadata could include the plasmid prep used, as well as the cell line info, author, date of production, and any analytical data associated with the protein.
Data and metadata are stored and indexed in Benchling and can be easily accessed through search, REST APIs, or the data warehouse.
Accessibility
For data to be accessible, it needs to be easily searchable by humans and computational systems, without requiring extensive knowledge about its creator or date of creation. Benchling enables entries to be accessed by authorized personnel using the unique identifier and HTTP URL (internet link). Benchling also conforms to the openAPI standard, which allows computational systems to discover and understand the capabilities of the service without access to source code or documentation.
Individual Benchling user accounts can be configured using a granular permission structure, which offers flexible customer-admin defined user roles, along with the ability to grant specific types of access to external users using the UI and API. These REST APIs communicate with the client by automatically using the HTTPS protocol for authentication and protection of data in transit.
Interoperability
To achieve interoperability, an informatics system must use broadly applicable vocabulary and ontologies. Benchling’s configurable data model is built with standard vocabulary and ontologies that are agreed upon prior to implementation, and can map to any scientific process. While Benchling encourages the use of industry standards that follow FAIR principles, customers are free to use their own vocabulary, to conform to standards applicable to their industry.
In Benchling, links are created between data and metadata resources. These links provide qualified references between the metadata, enriching researchers’ contextual knowledge about each piece of information. Users can click on any single element and instantly view the whole picture — author, antibody chain info, number of antibody lots, plasmid preps info, etc.
Reusability
When existing data can be leveraged to answer new questions, it becomes reusable. Benchling’s unified informatics platform makes data points reusable, by making it easy for organizations to link them to the context under which they were originally generated — such as the materials used, protocol used, date of generation, and experimental parameters.
Many scientific communities use the HELM standard to deal with many subtleties of biologics (antibodies, antibody fragments, oligos, etc.). Benchling aligns with this framework, allowing information to be easily shared among scientists and organizations across multiple disciplines.
The impact of FAIR principles with Benchling
FAIR data is essential to solving the inefficiencies that have arisen from legacy data management practices, such as data silos, inconsistent terminologies, and lack of sufficient context on the data.
Benchling is designed from the ground up to support FAIR data, and has an in-house professional services team who facilitate the smooth transition to FAIR principles throughout every stage of an organization’s journey. Adopting Benchling will enable the creation of a centralized and unified data foundation, which is becoming increasingly necessary for leading-edge life science discoveries.
Hundreds of customers report that Benchling’s cumulative value across several dimensions has helped them reach milestones quickly:
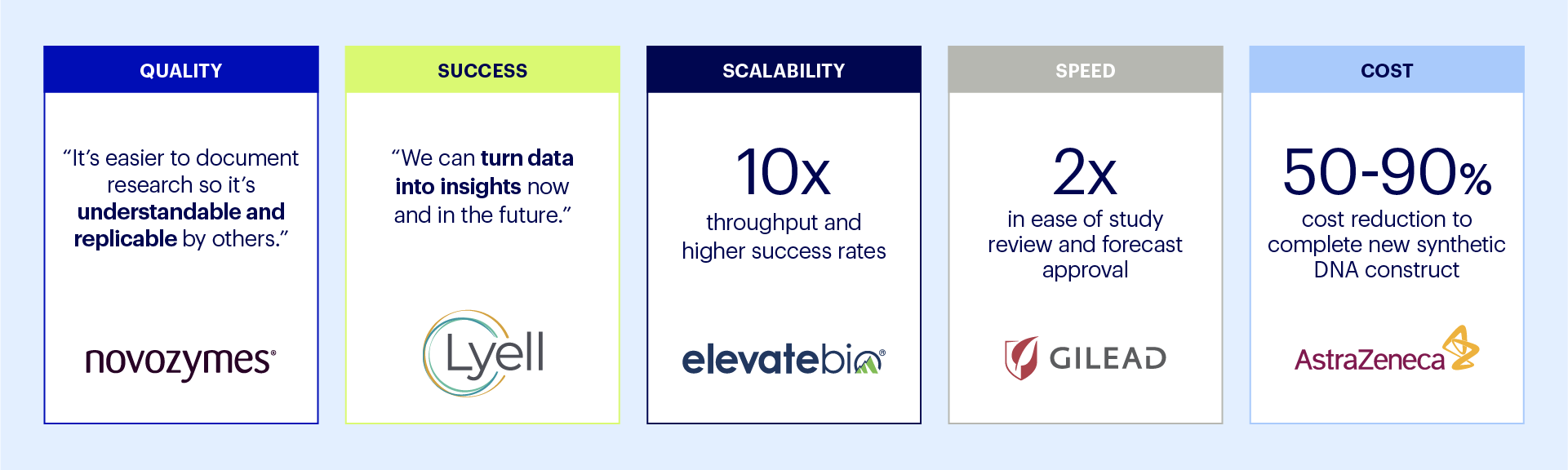
Read more on FAIR data principles in R&D
Strong data management has the potential to revolutionize decision-making, inform research and development, achieve tangible cost savings, and inspire innovation. But organizations can't access the full potential of their data without sound data governance. By aligning with FAIR principles, companies can bring products to market faster.
Read more on FAIR data principles in the one piece companies are missing in their R&D data strategy and how to establish a strong data strategy in R&D.
Powering breakthroughs for over 1,200 biotechnology companies, from startups to Fortune 500s