Lessons from Shape Therapeutics: How to harness AI for R&D success
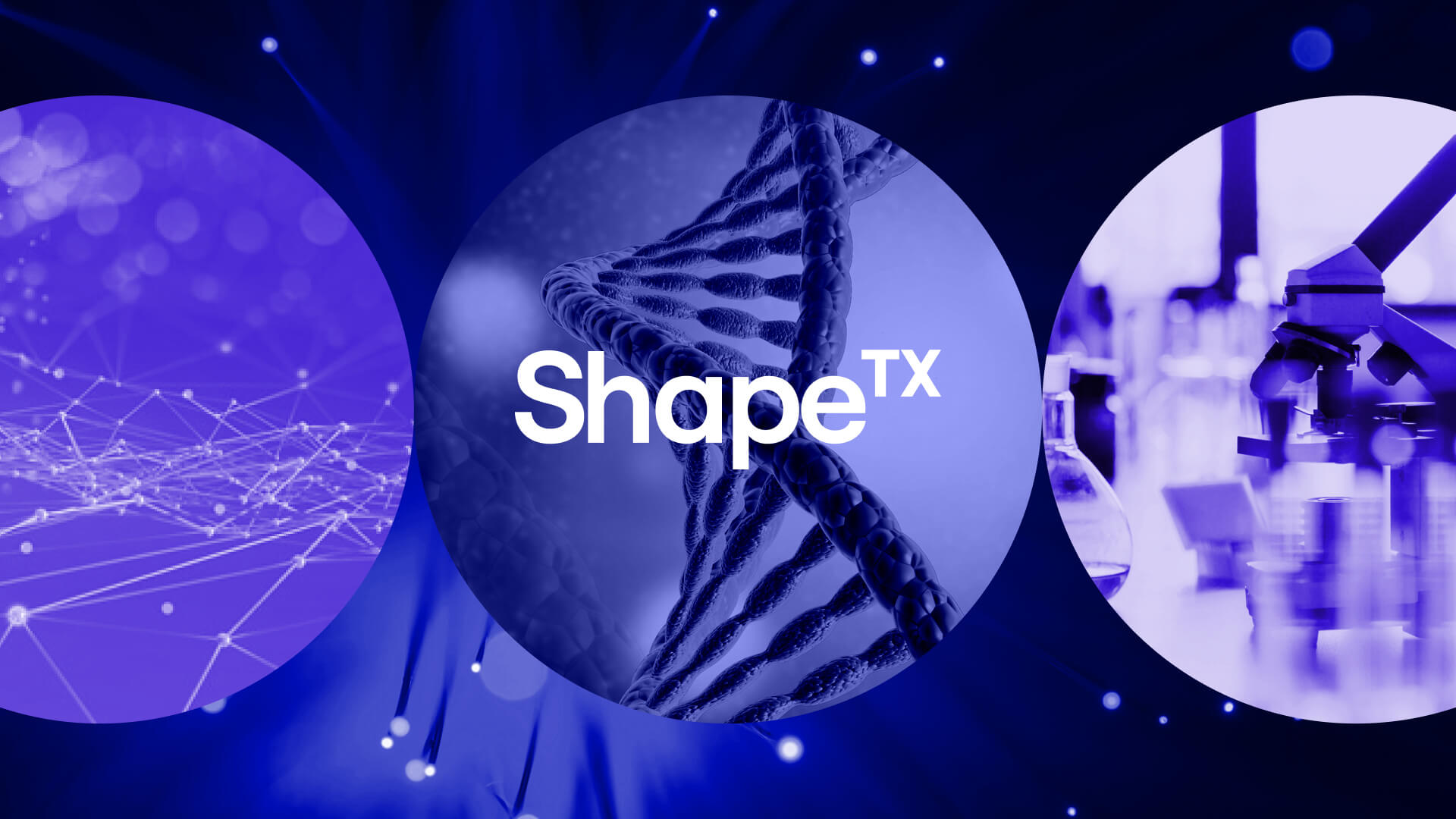
Scientific experimentation alone creates a slow R&D cycle. But five to ten years ago, there were few other viable options. Today, it's clear we've entered a new era of R&D that looks radically different.
For example, at Shape Therapeutics, we’re developing programmable RNA therapeutics that take advantage of existing human proteins that already edit RNA in the body — opening up the possibility of single-dose, curative treatments for thousands of diseases. However, when designing guide RNAs to ensure specific, targeted edits, there are on the order of 1043 possible sequences. Add in capsid engineering for improved delivery (~10956956 solutions) and gene regulatory elements for controlled expression (~10102
solutions), and the solution space becomes prohibitively large for experimental sampling to be effective.
That’s where generative AI comes in. We need computational power to help extract meaningful insights from our high-throughput experimental datasets. From there, we go back to the wet lab to test AI-designed candidates, using that experimental data to further refine and reinforce our AI models. At ShapeTX, collaboration between the wet lab and dry lab is essential — but requires a fundamental shift from the way R&D is traditionally done.
ShapeTX joined Benchling on BIO to talk about what it means to have AI-ready data, AI-enabled scientists, and how we’re approaching all of this at ShapeTX. Here are some of our biggest learnings.
1. High quality data is more important than AI architecture
While it’s become relatively straightforward to implement AI, the key differentiator between companies is having enough high quality experimental data to train their models.
To have truly AI-ready data, your data must first be findable and accessible — it can’t be stuck on desktops or hard drives. Data should be centralized and captured in a way that prevents data silos, while maintaining data integrity.
Next, it’s not enough to just have data. You need data and metadata to effectively train your models. Metadata provides your models context on relevant variables that explain their predictions, preventing incorrect conclusions due to factors like batch effects.
Finally, your data should be standardized. Integrating data from dozens of lab instruments — all with their own proprietary data formats — remains a major challenge, even at ShapeTX. Data ingestion becomes complicated, especially when you consider all the data coming from PDFs that aren’t machine readable. Having a consistent data format helps your ML models train more easily, because they’ll already understand the structure of the data they’re looking at.
Moving towards an open source standardized data format that’s established across the field will improve AI and ML applications across biotech. Benchling's new initiative with the Allotrope Foundation is a major step in the right direction for the entire industry. Check out their open source library for converting instrument data to a common format.
2. Everyone needs to see how AI is enhancing their work
Ensuring AI success is a team effort. The entire R&D organization needs to understand and derive value from AI-driven approaches. Having AI-ready data starts in the wet lab, with the scientists at the bench.
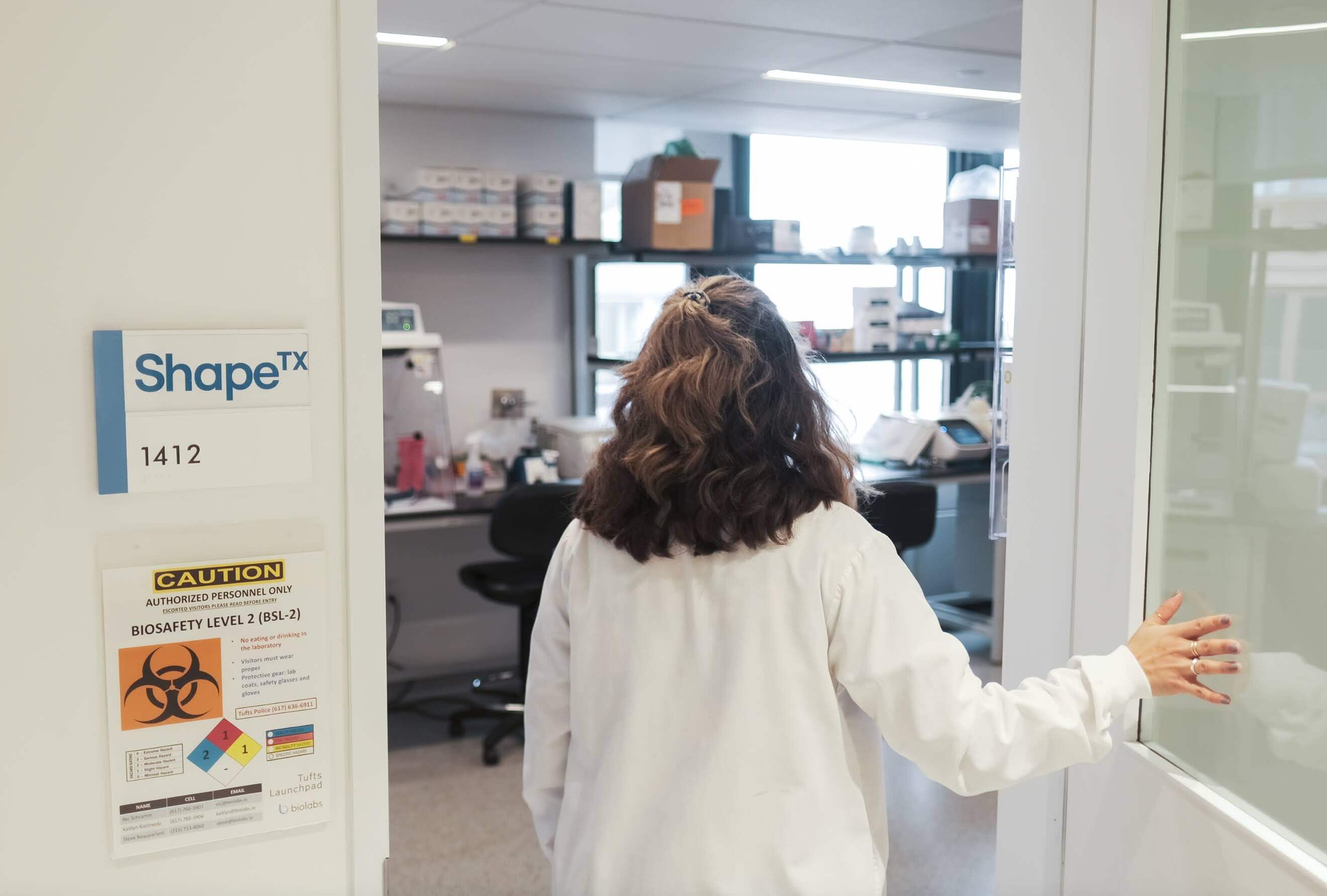
At ShapeTX, we give our experimentalists access to our models, so they have a better idea of how the system works. By demonstrating the value gained from AI — and why high quality data is so critical — our team understands why consistent data collection practices matter.
For us, setting up automated data pipelines that funnel data directly to our models was a key moment to not only drive immediate value for the team, but also highlight the power of automation.
3. Successful teams bridge the gap between lab scientists and data scientists
The key to AI success is collaboration between the wet lab and dry lab. Both sides need to work together, and learn from each other, to cultivate a new, hybrid approach.
Teams that skew too much towards experimentalists are in danger of applying basic, suboptimal AI approaches to their biological datasets — which was the industry norm five to ten years ago. In contrast, over indexing on AI can result in theoretical models without much biological relevance. Striking the right balance takes active collaboration from both sides.
At ShapeTX, we teach our experimentalists coding basics (e.g. Python, R), so they can look at their data and understand it. On the computational side, our data scientists need to understand wet lab processes enough to use the data properly. Having a hybrid team, with both wet and dry lab expertise, empowers organizations to pick the best ML approaches for their biological questions.
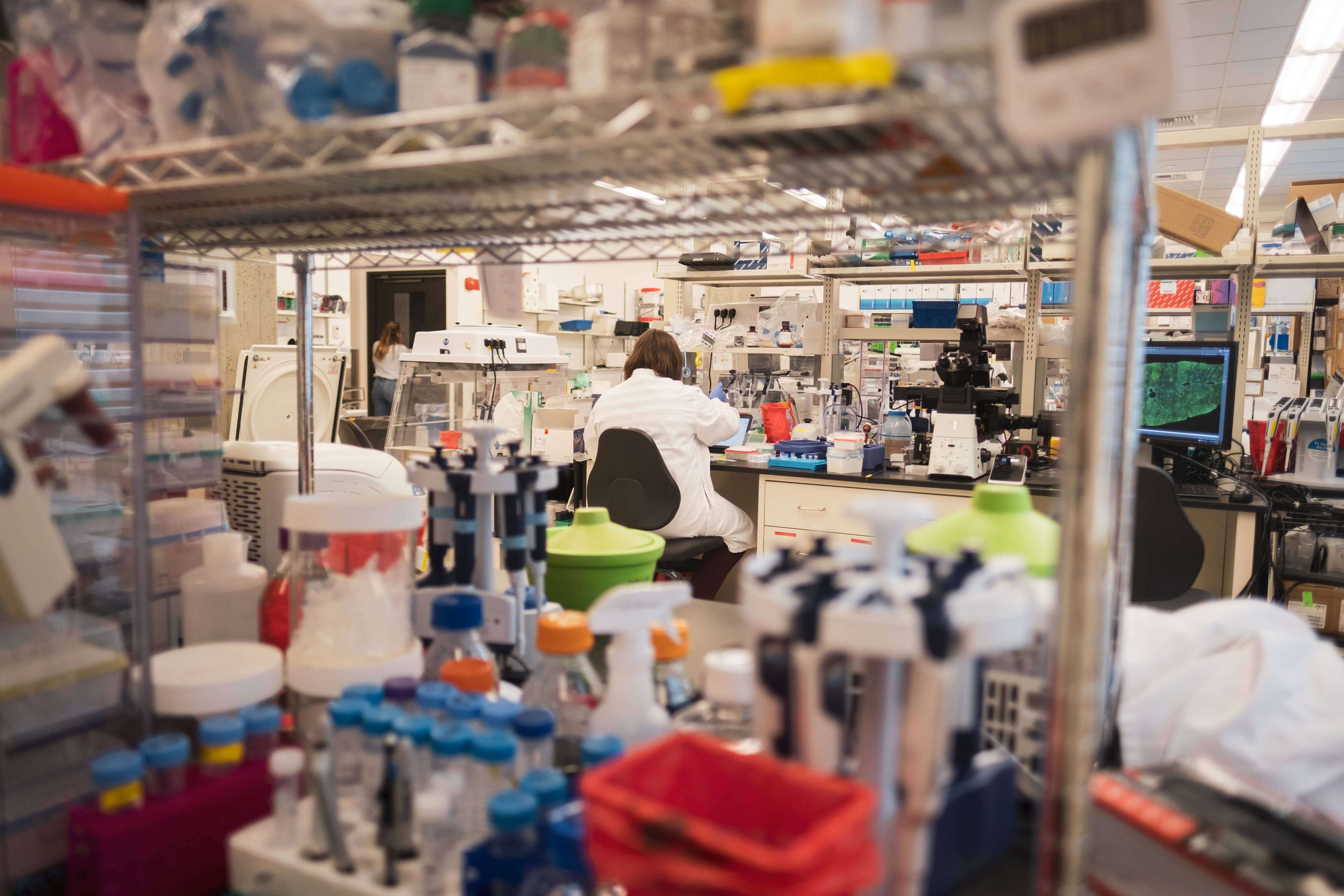
Beyond that, our AI team includes an array of data scientists, ML engineers, computational biologists, bioinformaticians, and IT professionals. By combining expertise across multiple domains within a single group, we can scale and secure our processes better than if we were working in isolation.
4. It’s not all about AI — the loop between the wet and dry lab is where the magic happens
Everyone may be thinking about AI now, but without the underlying experimental and preclinical systems to test, validate, and reinforce ML models, success remains challenging. High quality, standardized data must flow seamlessly between the wet and dry labs.
At ShapeTX, we use experimental data to both train and subsequently reinforce our models, as we identify AI-generated candidates and advance them towards the clinic. Working together as a team of wet and dry lab scientists is essential, and building a robust data foundation to support seamless data handoffs and collaboration is a critical first step for R&D teams.
AI is changing R&D, and we need to keep up
With AI, we’re no longer constrained by the practical limits of scientific experimentation. Now, we can generate and analyze billions of therapeutic candidates in silico before advancing the most promising ones to the wet lab.
We’re operating at an entirely different scale than was ever before feasible with traditional methods alone. But first — we need to scale our R&D processes and teams to match. For us at ShapeTX, that means the automation and data infrastructure to enable generation of AI-ready data, along with seamless data handoff and collaboration between the wet and dry labs.
Scientific discovery is often incremental, but every so often you see technologies that catalyze explosive progress. Biotech as an industry is at a pivotal point, with the potential to unlock orders of magnitude more breakthroughs. AI can fundamentally change the pace of tomorrow’s science — but only if we reinvent the way we do science today.
Download our guide on AI for biotech
Read more on getting started with LLMs in our guide to generative AI for biotech.
Powering breakthroughs for over 1,200 biotechnology companies, from startups to Fortune 500s