How Benchling is powering an open source approach to data standardization with Allotrope
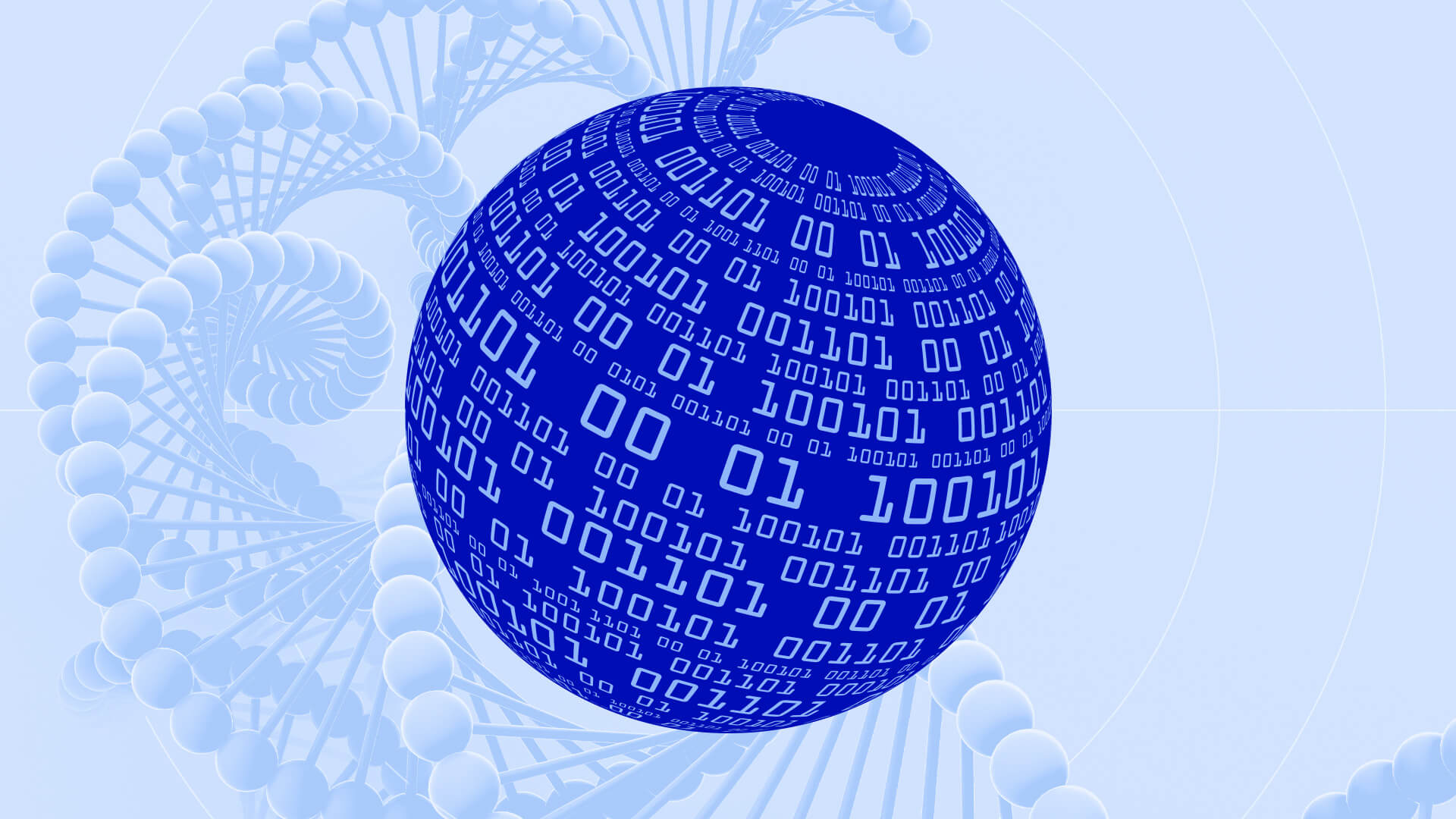
Earlier this year, Allotrope launched publicly available data standards using the Allotrope Simple Model (ASM). As an Allotrope partner, we want to take the next step to help put those standards into action. This past November, Benchling had the opportunity to present at Allotrope Connect, a two day event dedicated to discussing innovations in the standardization of data. We took the stage to share the power of an open source approach to data standardization and lab instrument connectivity. Read on to learn about Benchling’s strategy for powering data standardization in the lab, some of the hurdles we've encountered along the way, and how we’re working to make open source a reality.
The persistent data challenge
Better data leads to better science. Improved data management gives R&D and IT teams the ability to understand and compare data across with, or across, experiments. Leaders across the biology and technology industries can agree that building a strong data foundation is key to accelerating R&D. What they can also agree on is that doing so remains a persistent challenge that hasn’t yet been solved.
In Benchling’s recent State of Tech in Biopharma Report, we surveyed 300 biopharma experts to surface patterns around usage, impact, and barriers biopharma faces in adopting tech. Of those surveyed, 60% connect less than half of their instruments, resulting in data inaccessibility and fragmented analytics. The truth is in the numbers — something has to change.
Better data requires an open source approach
In biopharma, data is historically difficult to capture, manage, and engage with across teams within an organization. Companies are prioritizing FAIR data principles to allow for innovation, transparency, and efficiency. But with a proliferation of instruments, tools, and systems in modern labs, it’s difficult to actually make these happen in practice. In fact, in our survey, the majority of biopharmas reported that FAIR data principles are still out of reach, with notably limited progress in achieving organization-wide data interoperability at 28%, and data reusability at 30%.
In looking at the end-to-end instrument connectivity process, we see an opportunity to broaden the adoption of standardized data by open sourcing data converters.
Making open source a reality
Benchling launched an initiative last spring to standardize instrument data in the scientific community. As part of this initiative, we launched Benchling's Allotropy Python Library, a GitHub library of open source converters that format instrument-generated data to the ASM.
We launched this repository to fuel adoption of the ASM data standard. By making these converters available in the open source, companies of all sizes will be able to easily standardize and connect instrument data within and across their labs.
The process of creating this open source library wasn’t linear. Through this initiative, we’ve surfaced a number of key hurdles that have helped contribute to the development of our solution. Below are a few of the lessons learned.
1. Knowledge of Allotrope as an open standard
Let’s start by debunking some myths about Allotrope. The one we hear most commonly is the belief that ASM schemas live behind a paywall. While this may seem too good to be true, ASM schemas are open to the public! Not only that, they’re free for both commercial and non-commercial use.
2. Unique needs for research and IT / data scientists
While instrument data standards serve a variety of teams, we’ve found there to be a set of discrete needs and solutions for different biotech personas.
When it comes to the role of an IT or data scientist, these individuals need structured data to build analyses, as well as integrations with generic tools. Formatting that is vendor and technique agnostic is a requirement for solving the proliferation of data formats across lab instruments. This proliferation requires lab IT scientists to encounter and solve for data conversion again and again, slowing down productivity. To solve this lack of knowledge of experiments and technique, the right solution should be self-documenting. Because biotech is always changing, adaptability for future use cases is vital.
Research scientists have their own needs — they’re often looking for the ability to capture data from technique specific analytical tools. This data needs to be expressed in a familiar ontology, and just as with their IT counterparts, interpretable and actionable now and in the future.
To address the needs of both IT and research scientists, a Calculated Data Document is now part of the ASM core schema, and has already been incorporated in the qPCR and dPCR models. For IT and data scientists, a large benefit is that the data source identifier allows for associating relationships between calculated values. The inclusion of a Calculated Data Document segregates ‘ground-truth’ measurement records from calculated results, facilitating parsing for re-analysis. For research scientists, this release provides a means of capturing calculated results returned by instrument or defined by user. By allowing for recording information that is immediately actionable — without need for further analysis — insights can make it to market faster.
3. Enabling implementation of the standard
An open standard needs an open means of implementation. To accomplish this, a standard must not only be defined, it must also be maintained. It’s important to note that the process of defining a standard and the process of implementing a standard are, and should be, separate activities.
Without implementation, the standard cannot achieve its intended benefits. While self implementation is an option, it possesses scalability limits, and often results in duplicative effort. When it comes to organization-wide usage, it can be harder to gain adoption from stakeholders who do not benefit directly from standardization.
Connecting the standard to the lab
To put this all into practice, it takes a collaborative, team effort. By combining the power of Allotrope, Benchling's Allotropy Python Library, and Benchling, the standard can now come to life within the lab. Here’s a bit more about how this partnership achieves that outcome.
Allotrope: Allotrope is on a mission to revolutionize the way we acquire, share, and gain insights from scientific data. It serves to reason then that Allotrope plays a critical role in maintaining ontology and data models. To bring the standard to the lab, Allotrope publishes schema for modeling instrument data, and provides governance of the standard.
Benchling's Allotropy Python Library: The library provides open source tools for converting data to ASM, and is published under an MIT license. Maintained by Benchling (with community contribution welcome!), the Allotropy Python Library is available for anyone to use and implement.
Benchling Connect: Benchling Connect is a service for exchanging data between instruments and Benchling. Using our Allotropy Python Library for harmonizing instrument data, it enables labs to easily benefit from the Allotrope standard. If you’d like to see how Benchling Connect can help you standardize data, request a demo.
Learn more about data standardization with Allotrope and Benchling
Learn more about Benchling's vision for data standardization and our open source instrument data converters in our 2023 press release and Benchling Connect data sheet.
Powering breakthroughs for over 1,200 biotechnology companies, from startups to Fortune 500s