How to jumpstart innovation in R&D? Biotech must solve the instrument connectivity challenge
How to jumpstart innovation in R&D? Download your copy today.
There are several critical steps R&D companies need to power R&D innovation. We’ll cover the details of each below.
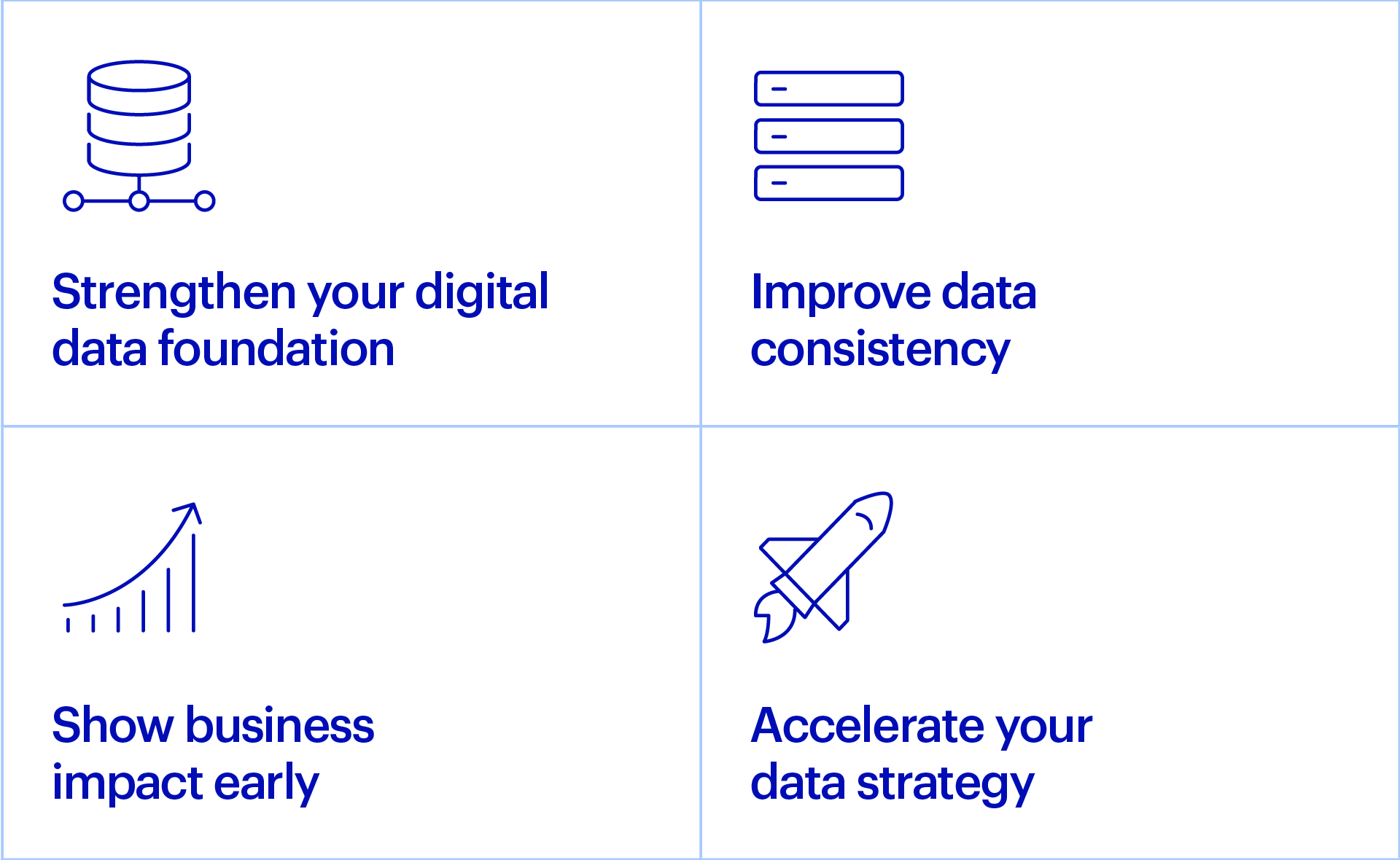
1. Strengthen your digital data foundation
Develop a strategy that strengthens your digital data foundation and scales your data infrastructure. A robust data infrastructure facilitates innovation by providing R&D teams with access to historical data, insights, and tools that can inspire new solutions.
Key to achieving a stable, scalable foundation is investment in a data lake or data warehouse. In Benchling’s State of Tech in Biopharma report, R&D data platforms were found to be the highest adopted enabling tech, with 70% reporting housing R&D data to be the reason.
While scalability is important, however, flexibility is equally important to consider — especially as organizations experiment early on. More than half (54%) of those who adopted a data platform reported that their solution was custom-built, and a further 74% of those cited use of AWS, Microsoft Azure, Google Cloud Platform, Snowflake, or Databricks.
Software that can evolve with your data will future proof your organization, as will software that aids in centralizing your data. Creating a single location in which all data is stored will ensure clarity, accuracy, and accessibility, while avoiding potential errors, data loss, or discrepancies
2. Improve data consistency
Developing a central source of truth to house your organization’s data means teams will no longer need to rely upon a disparate collection of systems. This not only eliminates the inefficiencies associated with switching between multiple tools, it simultaneously improves collaboration by ensuring everyone is communicating using the same accurate information. To establish connections between data sets and improve lab instrument data management, companies need to adopt universal data standards. By adopting data standards like Allotrope Simple Model or open JSON model across teams, instruments, and systems, teams can improve data consistency, and ease of use.
There’s been a mindset shift. If you want to centralize all your data assets and be able to quickly incorporate new ones, since the fundamental idea is we have data from different sources, we need to organize, normalize, and apply governance to make it accessible and accelerate discovery. - Aaron Friedman, Principal Startup Solutions Architect, Healthcare and Life Sciences, Amazon Web Services
3. Show business impact early
According to a recent Accenture survey of 250 executives, 60% of early digital adopters have not been able to measure success from the transformation thus far. While this doesn’t necessarily mean that success isn’t happening, it does point to a universal challenge for R&D companies: showing business impact.
Measuring business impact can help justify the investment required for new technologies, and further encourage adoption across the organization. By starting implementation within a single team or department, you gain the opportunity to iterate on your data strategy and define a repeatable model. Once the success of this model has been validated and proven, achieving scale across the company is much more attainable.
From productivity and collaboration improvements, to cost savings and better insights, many business benefits are possible. When going through the process of determining value metrics, consider the downstream effect of short term benefits that can have long term impact on your organization.
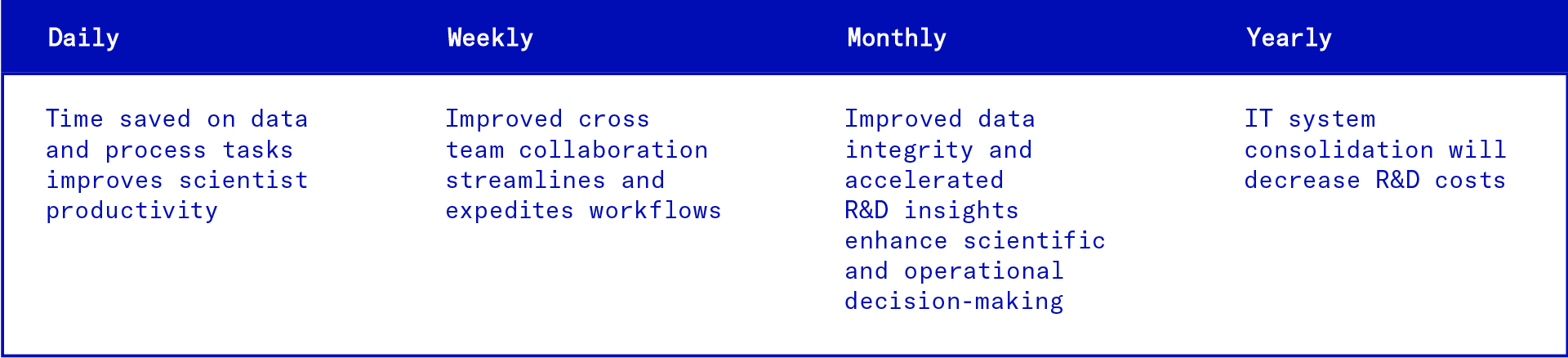
The culmination of these benefits help shorten time to milestone and get the right drug into the clinic.
4. Accelerate your data strategy
Establishing a scalable data infrastructure, ensuring data consistency, and showing early business impact are all foundational to improving lab instrument connectivity. To take your data strategy to the next level, however, consider integrating out-of-the-box, configurable solutions.
As teams scale, so does the number of instruments and tools, necessitating custom integration and ongoing data management. With Benchling Connect, companies can achieve powerful lab instrument connectivity and more accurate end-to-end lab instrument data management, using simple file-based or API-based integrations.
Today, Benchling Connect supports out-of-the-box integrations with 12 commonly used instruments. Connect allows teams to easily standardize data and prevent vendor lock-in by mapping all instrument output to the Allotrope Simple Model (ASM), and making the converter codes open source and freely available on GitHub.
Solving the foundational problems of your data infrastructure early on is also imperative for successful incorporation of AI and ML. When it comes to large language models (LLMs), investing in a data strategy that ensures consistency, structure, and scalability opens up a whole realm of possibilities for LLMs across the R&D lifecycle.
Data connectivity is critical to the future success of R&D organizations. Though life science and biopharma companies are lagging behind when it comes to digital data maturity, laboratory instrument connectivity offers an opportunity to realize the value of your data. Modern R&D requires a strong digital data foundation informed by standardization, consistency, and scalability. By establishing a data strategy that supports connectivity, teams are best positioned to embrace the future of science.
Ready to take the next step to power biotech innovation? Learn more about how your company can improve lab instrument connectivity with Benchling Connect.
Powering breakthroughs for over 1,200 biotechnology companies, from startups to Fortune 500s