Data-driven biological research: A biotech’s guide to establishing a strong data strategy
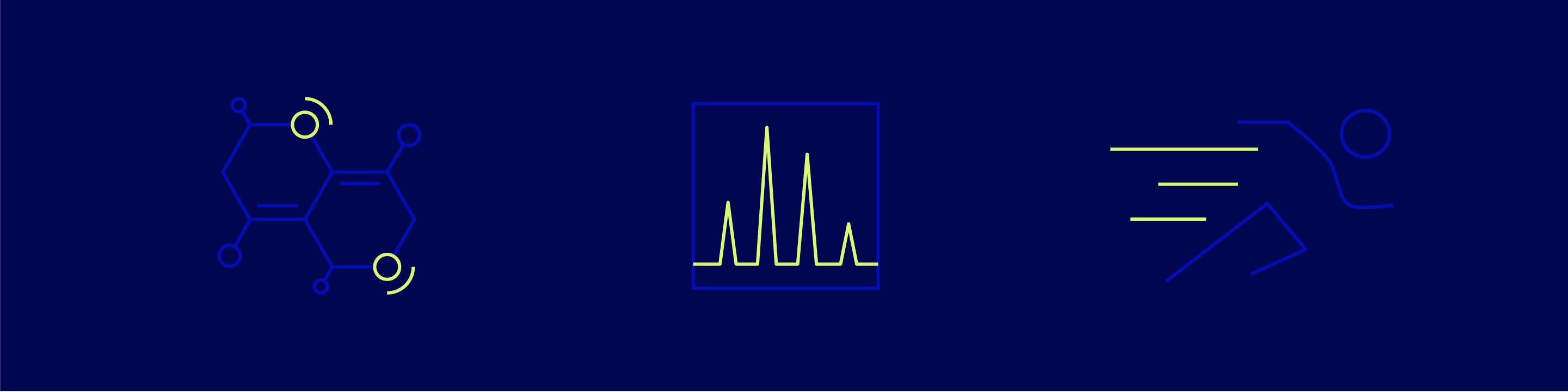
Identifying and addressing the top data challenges in research and discovery
Science is becoming more sophisticated and complex. What was once a simple molecule drawn on a whiteboard is now a complex protein whose structure must be represented with a multi-dimensional model. Whole genomes are sequenced in as little as a day. The most minute changes in cells can be observed through advanced microscopy.
All of these shifts point to the undeniable fact that we are in the midst of the most important scientific revolution since computing. And if you’re in biotech, you’re driving this revolution. Solving the world’s most complex societal problems — infectious and genetic diseases, feeding a growing population, solving the climate crisis — is precisely what biotech companies are positioned to achieve. But doing so requires continuous innovation.
Today, research and discovery is more nuanced than ever, requiring sophisticated, seamless knowledge transfer across teams, from finding new biomolecules to drug development and regulatory submissions. Most notably, it requires those teams to rely heavily on any biotech company’s most valuable asset: their data.
In this article, we cover key challenges biotech companies are facing with data, plus a strategy for approaching building a FAIR data strategy.
Science is increasingly complex — and data is growing exponentially
There are several trends taking place today that will fundamentally change the role data plays in accelerating the pace of scientific innovation.
1. New modalities
New molecule types and scientific techniques are being introduced more regularly. Much of the drug discovery pipeline has shifted from predominantly small molecules to an even number of NMEs and biologics, requiring a new multi-dimensional data model.
2. Data sets are growing and increasingly complex
In addition to the growing range of modalities, scientific data sets themselves are growing in size and complexity. Biotech data, largely fueled by genomics data, is doubling every 7 months in size, making biotech the industry with the largest data volume by 2025.
3. Expectations for speed are increasing
In the face of these complexities, the industry is facing pressure to get products to market faster than ever before. COVID vaccines and new cell and gene therapy products, for example, have proven that cutting-edge therapies can get from discovery into the clinic in as little as two years. This has fundamentally changed the goalposts for companies trying to launch new products.
Despite the growing complexity of science and scale of scientific data, the way scientists do their work has not meaningfully changed:
Many labs are still using paper, Excel, or PowerPoint to document, research, and track projects.
For those who have made the move to digital systems, the legacy software they rely on wasn’t designed to handle the complexity of biologics and modern techniques.
Historical data is languishing in data silos where it can’t be easily accessed or used for current and future experiments.
What makes FAIR data so difficult in R&D?
In 2016, the journal Scientific Data launched FAIR data guiding principles — standards which assert that data is Findable, Accessible, Interoperable, and Reusable. The goal with FAIR data principles is to allow people and computers to collectively gain the most value from data, whether it’s public or private, no matter the source it’s coming from.
Upholding FAIR data standards is a significant challenge for biotech companies, who are managing a vast range of data types, large datasets collected from disconnected sources, and working across many different research teams.
Benchling surveyed over 600 scientists on their top pain points when it comes to data. We found that teams are working to overcome several compounding, universal challenges:
1. Upkeep of data across systems is time-consuming manual work for scientists and R&D IT.
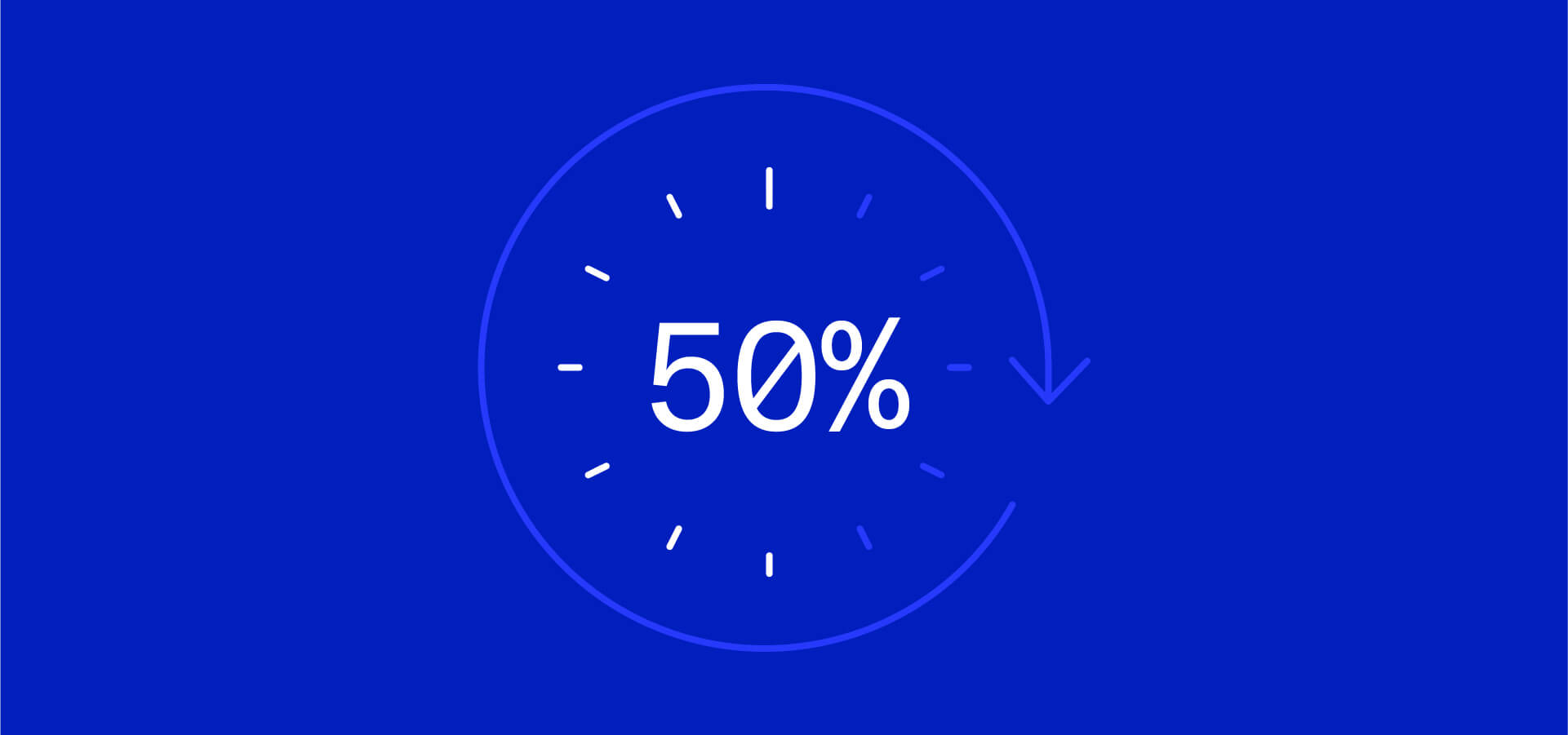
The proliferation of applications — such as multiple legacy ELN, LIMS, LES, and MES systems — equates to serious cost for scientists. Scientists spend nearly 50% of their week doing basic data entry and other process-related tasks when using legacy solutions.
2. Sharing data is difficult.
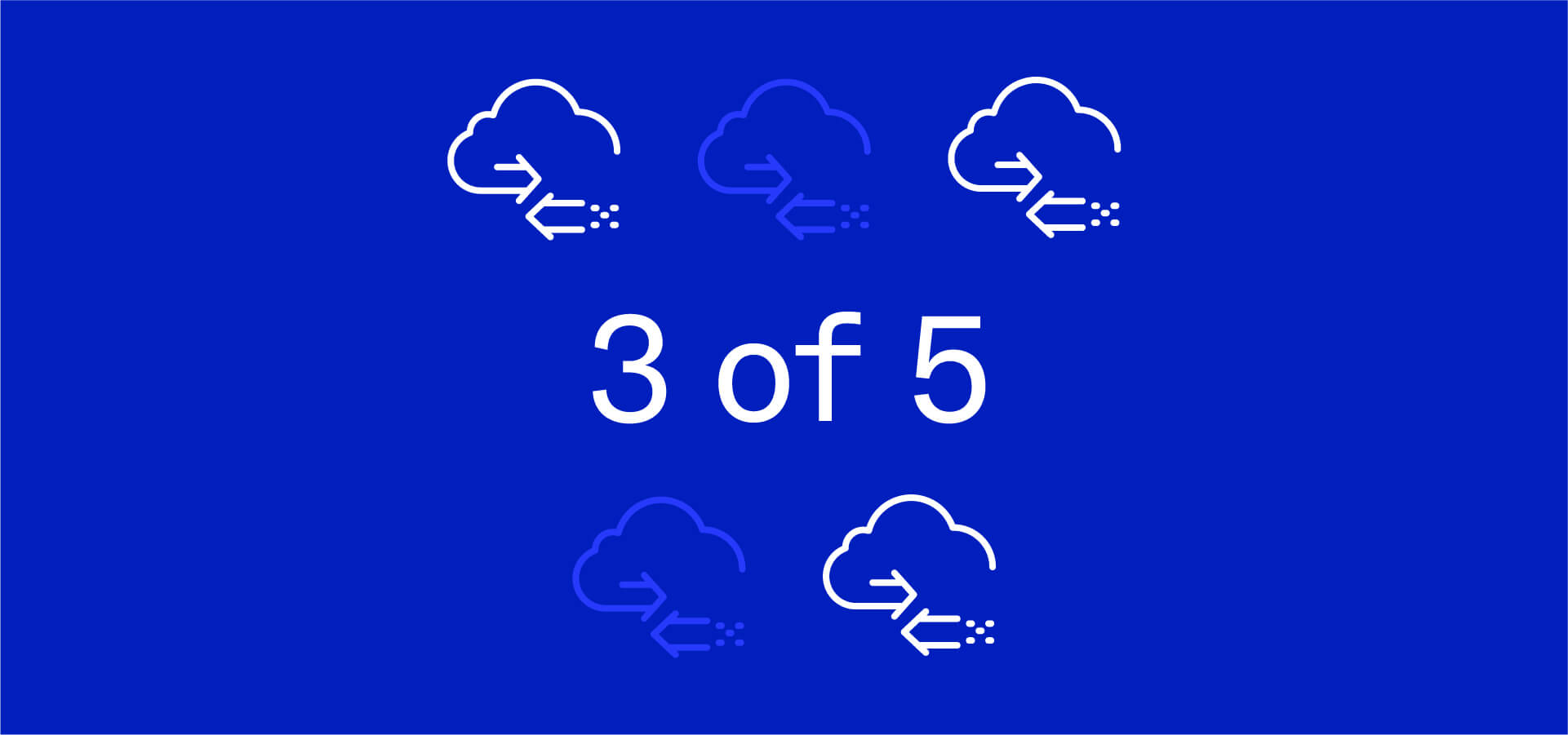
This drag on productivity doesn’t only slow scientists down; it also impacts other teams — a fragmented ecosystem poses real barriers to real-time collaboration. On average, those surveyed reported 3-5 handoffs any time data is passed between teams, causing time, data loss, and friction as data moves from research to development.
3. Confidence in data accuracy is low.
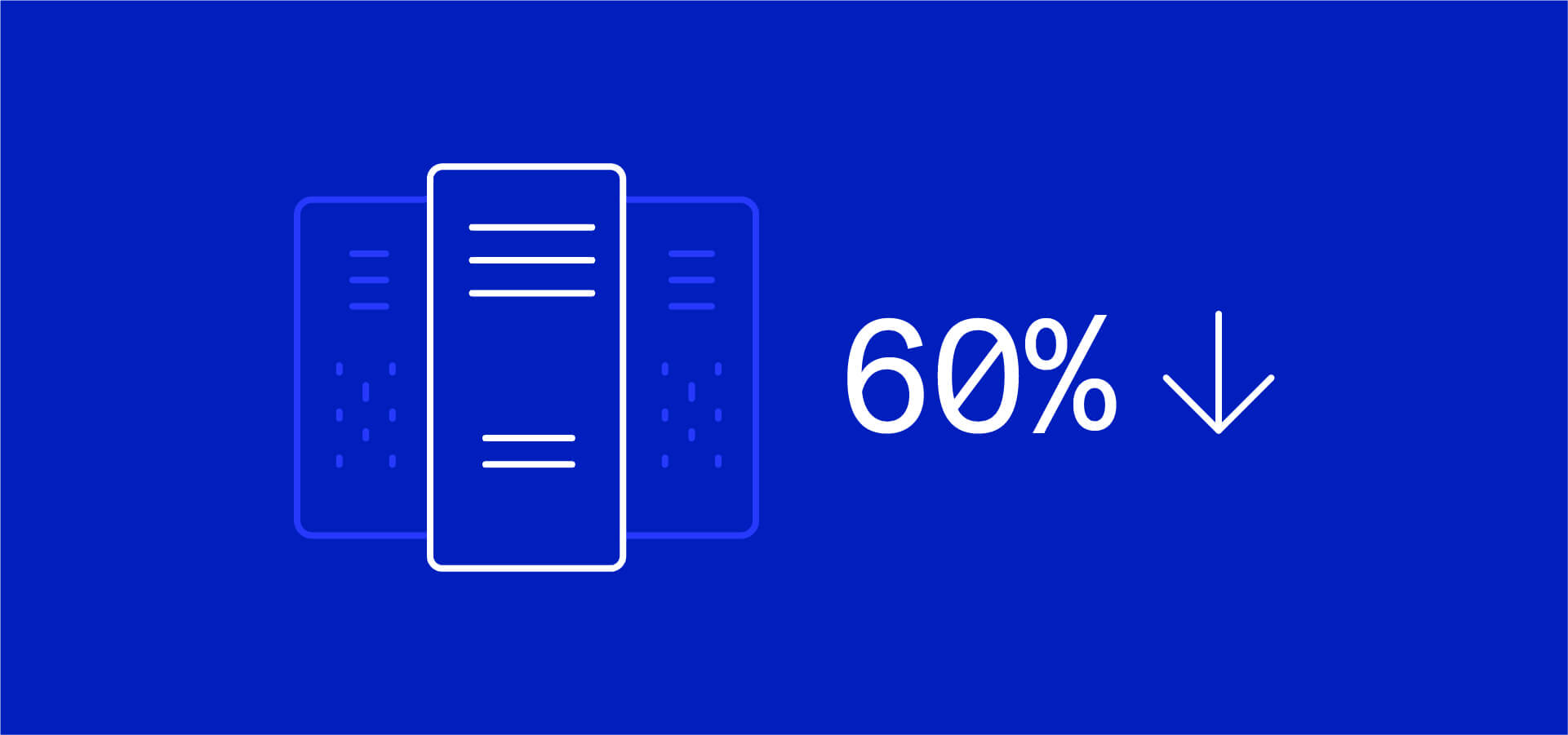
With a limited ability to find and access data, and the reality that data is often entered manually in an unstructured way, scientists reported only 60% confidence in data accuracy, and half of scientists reported they struggle to access the insights they need to do their work.
A 3-pronged approach to FAIR data transformation
There are several critical steps R&D companies need to consider when adopting a FAIR data strategy:
defining data architecture
determining how data will be governed and stewarded
using the hub-and-spoke model
We’ll cover the details of each below.
1. Define your data architecture
To begin rethinking your data architecture, and more specifically, how you want to capture and manage your data to ensure it gets used by multiple teams across the R&D continuum, you must outline your current approach to data.
How many disparate solutions do you use for data management?
What solutions are you using to collect, house, and access data?
How integrated are these to support data capture, search, access, and analysis?
Are you easily able to update and iterate on your existing data models?
What types of data are you collecting right now, and is there data you should be collecting that you’re not?
Once you establish a baseline of your current data maturity and the limitations you face in capturing and accessing data, you can define requirements for your future goals.
2. Determine your data governance model and stewardship strategy
To determine the best data governance model for your organization, you first need to understand how teams across the business want to use the data you're capturing, and who will be accountable for ensuring the organization adheres to the model you choose.
What are the top priorities of specific R&D teams and groups within your organization?
Which data points are critical to their goals?
What types of data are needed for informed decision-making across the organization?
What types of data are needed for submitting to regulatory bodies?
Who needs to have access to different types of data?
Who is held responsible for enforcing the governance strategy?
Which teams and individuals own data entry and updating processes?
Who is responsible for ensuring data is mapped and modeled correctly when passed between teams?
When new technologies are introduced, who is in charge of ensuring that data is incorporated into the existing strategy?
3. Use a hub and spoke model
R&D companies can adopt a hub and spoke model to restructure data capture.
Legacy systems such as electronic lab notebooks and LIMS software create data silos within and across discovery and research. Since each system is used by different teams in different ways, data is stored in different places and isn’t standardized. As these systems proliferate — either via vendors or home grown — a scientist has to work across a jumble of systems and tools, or "spokes", that don’t interoperate with each other.
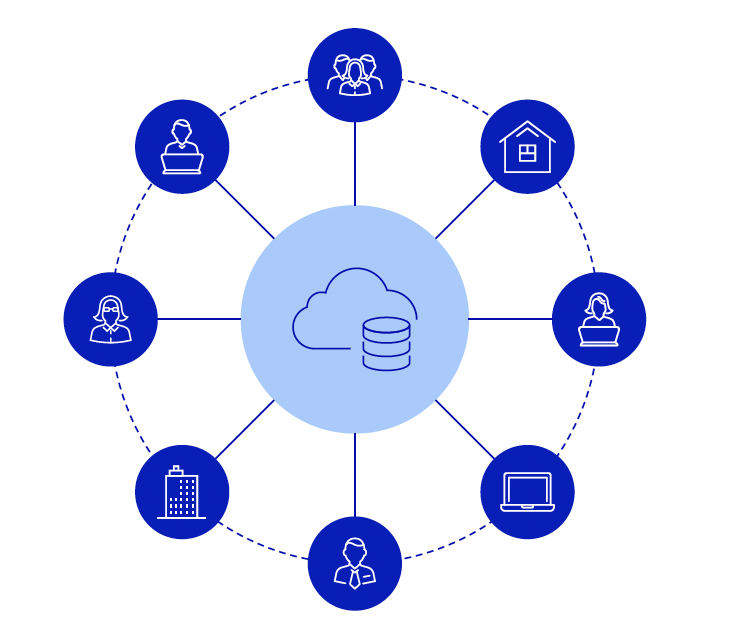
That’s where the hub comes in; it’s a tech solution at the center that houses the data from all the spokes. A hub and spoke model can provide scientists with a central source of truth or central interface to access data that exists across multiple systems. It allows companies to add data in an intuitive and structured way, so it can be reused and easily accessed by others across the organization.
For this model to work, you need technology that supports connections to other systems, rather than being standalone — such as having open APIs to allow for extensibility and direct connections to commonly-used third party tools, like instruments and analytics software such as Pluto.
The hub and spoke model is the approach we’ve taken with Benchling — giving teams a central place to connect disparate systems so they can access and engage with data.
The time is now for scientific data transformation
Voices across the biology and technology industry agree that building a strong data foundation is an urgent problem to solve in order to accelerate R&D. “The main barrier right now is data: we simply lack enough high-quality, well-annotated, consistent, and relevant data to solve meaningful problems in drug development,” said Jacob Oppenheim, PhD in Digitalis Press.
The strong data foundation is also critical for companies to be able to reap the benefits of new technologies down the line, according to Benchling’s Software Engineer Janet Matsen: “As un-sexy as it is, companies need to build their data strategy and systems before benefitting from machine learning. Beyond simply collecting data, doing so in a standardized way and anticipating how the data will be consumed, is key. Companies need to design their data systems for the analytics and AI and ML they aim to layer on top.”
Read more about implementing AI and ML in our article Biotech is leaving AI opportunity on the table. Data and culture are the fix.
* This survey included results from 968 scientists across 41 Benchling customers. Metrics are quantified benefits from Benchling customers who reported improvements in specific areas. Baseline data was collected from prospects prior to implementation and from selections of customers post implementation, via end user surveys or interviews. The Benchling team worked with these customers to validate the improvements they realized over time.
Powering breakthroughs for over 1,200 biotechnology companies, from startups to Fortune 500s