3 principles for biotech IT change management: Lessons learned from thousands of implementations
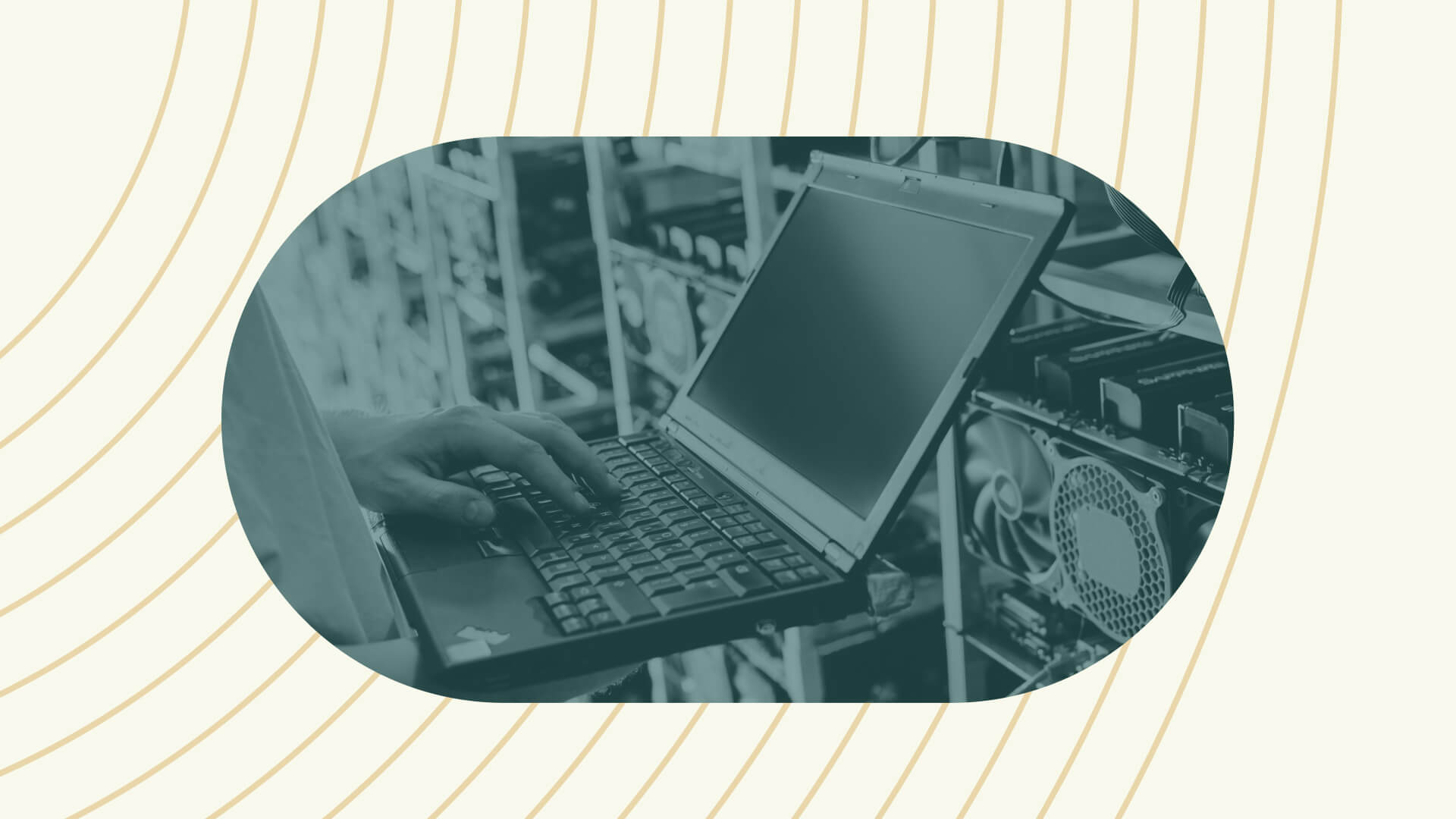
Change management is the number one barrier to successful technology adoption across all industries. 70% of transformations fail, not because of technical issues, but because leaders fail to address the increasing importance of managing change.
In biotech, IT and scientists are often out of sync when it comes to introducing new tools and sunsetting old ones. As a head of IT at a global pharma put it recently: "Scientists are stubborn. IT can be robotic. It's not always the most natural or productive pairing." While this challenge is certainly not exclusive to biotech, the techniques needed to improve IT change management in life sciences are unique.
After completing thousands of software rollouts across companies large and small, Benchling’s implementation team has developed a set of tried and tested best practices for smooth change management and new technology adoption.
1. Tie technology to a critical R&D outcome
Biotech companies often make the mistake of starting IT projects without taking the time to identify target outcomes. Not taking time to think about ROI misses an important opportunity for internal alignment: to take this belief in the power of technology and convert it into realistic goals to rally around; to make prioritization decisions; to encourage adoption; to drive change.
There are many possible outcomes to consider when defining R&D outcomes:
Speed: decreasing the time it takes to get to a milestone
Success: increasing probability that a candidate will be successful at the next stage
Scalability: increasing the throughput of experiments that can be performed
Data quality and integrity: increasing the quality of accessible data
Efficiency: reducing costs or driving efficiencies
While these categories all contribute to the bottom line, speed and success are often top-line goals for R&D executives, so they can drive IT change and investment.
When it comes to speed, measuring time to market or clinic can take many years and make it hard to tie IT changes to outcomes. Instead, using decrease in cycle time is a more near-term primary variable for measurement. For example, we support an antibody discovery organization with a goal of cutting time for a “learning cycle” in half (i.e. a cycle of trying out variants of a lead candidate and learning which ones work better). Software contributes to the overall strategy by reducing the time it takes to ingest and analyze data for selecting the optimal candidates.
Improvements to the probability of success are harder to measure. R&D leaders equate advancements in this area to better decisions about the candidate. As a result, qualitative measures that resonate with leaders are typically concerning new questions they can ask of their data to facilitate decision-making. One of our customers, an assay development organization, is focused on new cross-program questions they can ask of assay definitions and data. Could an assay be simplified by examining just one biomarker instead of multiple while achieving the same accuracy, for example?
These types of measurements, even if qualitative, support a greater focus on not only producing data, but more importantly, using data effectively — which is often the true ROI that R&D is looking for.
2. Start small
Scientists are cynics by training — a lofty vision isn’t going to win them over as much as showing incremental value. Achieving this gradual scale requires starting small, and the decision of where to start is critical to your initiative’s success.
Here are a few guiding principles to help determine your starting line:
Understand the patterns of adoption within your scientific groups
Whether we like it or not, we all have pockets of resistance in our organizations — groups that want to stick to paper notebooks or hold on to outdated custom-builds. Often the best way to invoke change is to start by finding a handful of early adopters across teams, and then work with these individuals to act as your internal advocates.
Map out the flow of data throughout your organization
Being mindful of your organization’s data flow, including people, processes, and tools, provides insight into where barriers and opportunities lie. For many organizations, there is a scientific group producing data that is critical to the rest of the organization. This data also tends to face the greatest challenges in reaching the right people. Identifying this pain point allows early intervention, as well as an avenue for showing immediate value to the rest of your organization.
3. Put R&D in the digital driver’s seat
A common mistake we see is placing too much emphasis on the initial launch, with limited attention to how it will adapt over time to scientific change. Science is unique in how rapidly it evolves, meaning that R&D, not just IT, needs to take ownership over digital initiatives. Being in the digital driver’s seat, however, requires a model that works not just during implementation, but for the years to come after it, or the software risks becoming obsolete.
Orienting around this model starts on the ground, by empowering scientists in the design of their software, particularly with regards to their data model. Bringing together wet lab scientists (data producers), dry lab or data scientists (data consumers), and IT on a recurring basis post-go-live ensures that the data model evolves as science does. Spending the time upfront to understand the configurability of your software systems is equally important, so that technology adapts alongside your data.
The need for ownership isn’t just isolated to power users — it extends to the top. Too often, sponsorship gets pushed down multiple levels in an organization, often by R&D leaders who believe that practitioners should be making all of the decisions. But digital initiatives are best positioned to thrive long-term with sponsorship from R&D executives as well.
There are three critical roles R&D leaders must fill as sponsors, before, during, and after implementation:
1. Align on and approve target business outcomes
As discussed above, deliberately defining outcomes is often critical to get your sponsor engaged. With their backing and buy-in, it’s easier to make the initiative a priority across the organization.
2. Create space for scientists to engage in digital initiatives
As year-end approaches, leadership’s priorities shift to short-term scientific work, favoring quick financial returns over long-term gains. Proactively establishing room for digital initiatives that set your company up for the future will ensure continued and sustained investment.
3. Connect the dots for how initiatives benefit teams
Tops-down communication from leadership is important for ensuring that scientists understand the value of improved data structure. By having more structured data in software systems, teams save valuable time performing a data search and can automate repeated parts of their day-to-day work.
Accelerate R&D with successful IT change management
For biotech companies to thrive in today’s digital-first world, they must successfully manage change and smoothly integrate new technology solutions. Whether it’s speed or probability of success you are looking to impact, aligning on where to start, what to achieve, and who to engage is critical to achieving R&D outcomes. To learn more about the benefits of working with Benchling experts on your implementation, read more about our Professional Services.
Powering breakthroughs for over 1,300 biotechnology companies, from startups to Fortune 500s